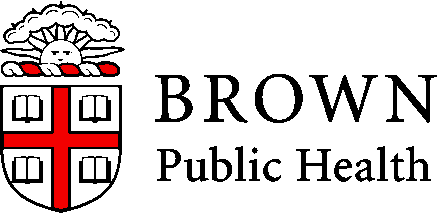
Adam J Sullivan
Assistant Professor of Biostatistics
Brown University
ggplot2
Appropriate graphical analysis may make the conclusions so clearcut that detailed specific analysis is unnecessary
-- David Cox (1978)
ggplot2
ggplot2
. ggplot2
do?ggplot2
cannot do:
ggvis
igraph
ggplot2
componentsggplot2
is built off the grammar of graphics with a very intuitive structure. ggplot2
will be more fluid and the more you learn about it the more amazing of graphics you can create. ggplot2
componentsggplot2
object:
geom_foo()
function. install.packages("fivethiryeight")
library(fivethirtyeight)
comic_characters
## # A tibble: 23,272 x 16
## publisher page_id name urlslug id align eye hair sex gsm
## <chr> <int> <chr> <chr> <chr> <ord> <chr> <chr> <chr> <chr>
## 1 Marvel 1678 Spid~ "\\/Sp~ Secr~ Good~ Haze~ Brow~ Male~ <NA>
## 2 Marvel 7139 Capt~ "\\/Ca~ Publ~ Good~ Blue~ Whit~ Male~ <NA>
## 3 Marvel 64786 "Wol~ "\\/Wo~ Publ~ <NA> Blue~ Blac~ Male~ <NA>
## 4 Marvel 1868 "Iro~ "\\/Ir~ Publ~ Good~ Blue~ Blac~ Male~ <NA>
## 5 Marvel 2460 Thor~ "\\/Th~ No D~ Good~ Blue~ Blon~ Male~ <NA>
## 6 Marvel 2458 Benj~ "\\/Be~ Publ~ Good~ Blue~ No H~ Male~ <NA>
## 7 Marvel 2166 Reed~ "\\/Re~ Publ~ Good~ Brow~ Brow~ Male~ <NA>
## 8 Marvel 1833 Hulk~ "\\/Hu~ Publ~ Good~ Brow~ Brow~ Male~ <NA>
## 9 Marvel 29481 Scot~ "\\/Sc~ Publ~ <NA> Brow~ Brow~ Male~ <NA>
## 10 Marvel 1837 Jona~ "\\/Jo~ Publ~ Good~ Blue~ Blon~ Male~ <NA>
## # ... with 23,262 more rows, and 6 more variables: alive <chr>,
## # appearances <int>, first_appearance <chr>, month <chr>, year <int>,
## # date <date>
names()
function:names(comic_characters)
## [1] "publisher" "page_id" "name"
## [4] "urlslug" "id" "align"
## [7] "eye" "hair" "sex"
## [10] "gsm" "alive" "appearances"
## [13] "first_appearance" "month" "year"
## [16] "date"
ggplot()
Basicslibrary(ggplot2)
ggplot(data=comic_characters, aes(x=align, y=appearances))
ggplot()
Basicsggplot()
Statementgeom_foo()
to make a proper graph. geom_point()
Statementgeom_point()
to this:ggplot(data=comic_characters, aes(x=align, y=appearances)) +
geom_point()
geom_point()
Statementggplot(data=comic_characters, aes(x=align, y=appearances)) +
geom_boxplot()
comic_characters <- comic_characters %>%
mutate(log_app = log(appearances))
ggplot(data=comic_characters, aes(x=align)) +
geom_bar()
ggplot(data= <DATA>, aes(x=<X-VARIABLE>, y=<Y-VARIABLE>)) +
<GEOM_FUNCTION>()
alpha
: makes points transparent to see overlaps betterfill
: Fills objects with color color
: Changes color of points or lines.shape
: Changes spape of pointsalpha
ggplot(data=comic_characters, aes(x=align, y=log_app)) +
geom_point(aes(alpha=1/100))
alpha
alpha
alpha=year
alpha
color
color
ggplot(data=comic_characters, aes(x=align, y=log_app)) +
geom_point(aes(color=publisher))
color
color="blue"
shapes
ggplot(data=comic_characters, aes(x=align, y=log_app)) +
geom_point(aes(shape=publisher))
shapes
fill
ggplot(data=comic_characters, aes(x=align)) +
geom_bar(aes(fill="blue"))
fill
color
fill
ggplot(data=comic_characters, aes(x=align)) +
geom_bar(aes(fill=publisher))
fill
geom_foo()
functions we can use. ggplot()
is a good place to start for more. To consider an example we will look at the data provided in the gapminder package:
library(gapminder)
head(gapminder)
## # A tibble: 6 x 6
## country continent year lifeExp pop gdpPercap
## <fct> <fct> <int> <dbl> <int> <dbl>
## 1 Afghanistan Asia 1952 28.8 8425333 779.
## 2 Afghanistan Asia 1957 30.3 9240934 821.
## 3 Afghanistan Asia 1962 32.0 10267083 853.
## 4 Afghanistan Asia 1967 34.0 11537966 836.
## 5 Afghanistan Asia 1972 36.1 13079460 740.
## 6 Afghanistan Asia 1977 38.4 14880372 786.
filter(select(gapminder, country, lifeExp, gdpPercap), country=="Kenya")
gapminder %>%
select(country, lifeExp, gdpPercap) %>%
filter(country=="Kenya")
gapminder
datacountry
, lifeExp
and gdpPercap
.# A tibble: 12 x 3
country lifeExp gdpPercap
<fctr> <dbl> <dbl>
1 Kenya 42.270 853.5409
2 Kenya 44.686 944.4383
3 Kenya 47.949 896.9664
4 Kenya 50.654 1056.7365
5 Kenya 53.559 1222.3600
6 Kenya 56.155 1267.6132
7 Kenya 58.766 1348.2258
8 Kenya 59.339 1361.9369
9 Kenya 59.285 1341.9217
10 Kenya 54.407 1360.4850
11 Kenya 50.992 1287.5147
12 Kenya 54.110 1463.2493
%>%
%>%
in the command you can think of this as saying then.gapminder %>%
select(country, lifeExp, gdpPercap) %>%
filter(country=="Kenya")
x1
and x2
. \[\sqrt{\sum(x_1-x_2)^2}\]
x1 <- 1:5; x2 <- 2:6
sqrt(sum((x1-x2)^2))
# chaining method
(x1-x2)^2 %>% sum() %>% sqrt()
x2
from x1
then we would sum those elementwise values then we would take the square root of the sum. # chaining method
(x1-x2)^2 %>% sum() %>% sqrt()
## [1] 2.236068