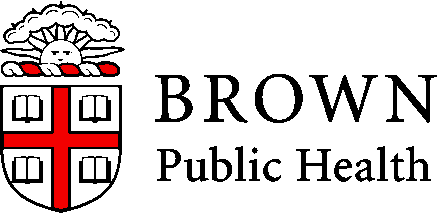
Adam J Sullivan
Assistant Professor of Biostatistics
Brown University
tidyr
The principles of tidy data provide a standard way to organize data values within a dataset.
--Hadley Wickham (2014)
spread()
Functionspread()
Functiontidyr
function we will look into is the spread()
function. spread()
it does similar to what you would expect.The picture below displays this:
## # A tibble: 12 x 4
## country year key value
## <fct> <int> <fct> <int>
## 1 Afghanistan 1999 cases 745
## 2 Afghanistan 1999 population 19987071
## 3 Afghanistan 2000 cases 2666
## 4 Afghanistan 2000 population 20595360
## 5 Brazil 1999 cases 37737
## 6 Brazil 1999 population 172006362
## 7 Brazil 2000 cases 80488
## 8 Brazil 2000 population 174504898
## 9 China 1999 cases 212258
## 10 China 1999 population 1272915272
## 11 China 2000 cases 213766
## 12 China 2000 population 1280428583
key
columnkey
, instead of there being values we see the following variable names:
## # A tibble: 6 x 4
## country year cases population
## <fct> <int> <int> <int>
## 1 Afghanistan 1999 745 19987071
## 2 Afghanistan 2000 2666 20595360
## 3 Brazil 1999 37737 172006362
## 4 Brazil 2000 80488 174504898
## 5 China 1999 212258 1272915272
## 6 China 2000 213766 1280428583
spread()
Functionspread()
function:spread()
Functionspread(data, key, value)
data
is your dataframe of interest. key
is the column whose values will become variable names. value
is the column where values will fill in under the new variables created from key
. data %>%
spread(key, value)
spread()
Example## # A tibble: 12 x 4
## country year key value
## <fct> <int> <fct> <int>
## 1 Afghanistan 1999 cases 745
## 2 Afghanistan 1999 population 19987071
## 3 Afghanistan 2000 cases 2666
## 4 Afghanistan 2000 population 20595360
## 5 Brazil 1999 cases 37737
## 6 Brazil 1999 population 172006362
## 7 Brazil 2000 cases 80488
## 8 Brazil 2000 population 174504898
## 9 China 1999 cases 212258
## 10 China 1999 population 1272915272
## 11 China 2000 cases 213766
## 12 China 2000 population 1280428583
spread()
Examplekey
in our spread()
function and value is the value
in our spread()
function.spread()
Exampletable2 %>%
spread(key,value)
## # A tibble: 6 x 4
## country year cases population
## <fct> <int> <int> <int>
## 1 Afghanistan 1999 745 19987071
## 2 Afghanistan 2000 2666 20595360
## 3 Brazil 1999 37737 172006362
## 4 Brazil 2000 80488 174504898
## 5 China 1999 212258 1272915272
## 6 China 2000 213766 1280428583
spread()
Examplecases
and a variable named population
. population
that is part of tidyverse. ## # A tibble: 1 x 3
## country year population
## <chr> <int> <int>
## 1 Afghanistan 1995 17586073
year
, assume that we wish to actually have each year as its own variable. spread()
function, redo this data so that each year is a variable. ## # A tibble: 219 x 20
## country `1995` `1996` `1997` `1998` `1999` `2000` `2001` `2002` `2003`
## <chr> <int> <int> <int> <int> <int> <int> <int> <int> <int>
## 1 Afghan~ 1.76e7 1.84e7 1.90e7 1.95e7 2.00e7 2.06e7 2.13e7 2.22e7 2.31e7
## 2 Albania 3.36e6 3.34e6 3.33e6 3.33e6 3.32e6 3.30e6 3.29e6 3.26e6 3.24e6
## 3 Algeria 2.93e7 2.98e7 3.03e7 3.08e7 3.13e7 3.17e7 3.22e7 3.26e7 3.30e7
## 4 Americ~ 5.29e4 5.39e4 5.49e4 5.59e4 5.68e4 5.75e4 5.82e4 5.87e4 5.91e4
## 5 Andorra 6.39e4 6.43e4 6.41e4 6.38e4 6.41e4 6.54e4 6.80e4 7.16e4 7.56e4
## 6 Angola 1.21e7 1.25e7 1.28e7 1.31e7 1.35e7 1.39e7 1.44e7 1.49e7 1.54e7
## 7 Anguil~ 9.81e3 1.01e4 1.03e4 1.05e4 1.08e4 1.11e4 1.14e4 1.17e4 1.20e4
## 8 Antigu~ 6.83e4 7.02e4 7.22e4 7.42e4 7.60e4 7.76e4 7.90e4 8.00e4 8.09e4
## 9 Argent~ 3.48e7 3.53e7 3.57e7 3.61e7 3.65e7 3.69e7 3.73e7 3.76e7 3.80e7
## 10 Armenia 3.22e6 3.17e6 3.14e6 3.11e6 3.09e6 3.08e6 3.06e6 3.05e6 3.04e6
## # ... with 209 more rows, and 10 more variables: `2004` <int>,
## # `2005` <int>, `2006` <int>, `2007` <int>, `2008` <int>, `2009` <int>,
## # `2010` <int>, `2011` <int>, `2012` <int>, `2013` <int>
gather()
Functiongather()
Functiontidyr
function we will look into is the gather()
function. gather()
it may not be clear what exactly is going on, but in this case we actually have a lot of column names the represent what we would like to have as data values. gather()
Function Examplespread()
practice you created a data frame where variable names were individual years. table4
:## # A tibble: 3 x 3
## country `1999` `2000`
## <fct> <int> <int>
## 1 Afghanistan 745 2666
## 2 Brazil 37737 80488
## 3 China 212258 213766
spread()
practice.year
is a variable and 1999 and 2000 become values instead of variables. gather()
Functiongather(data, key, value, ...)
data
is the data frame you are working with. key
is the name of the key
column to create.value
is the name of the value
column to create....
is a way to specify what columns to gather from. gather()
Exampletable4 %>%
gather("year", "cases", 2:3)
## # A tibble: 6 x 3
## country year cases
## <fct> <chr> <int>
## 1 Afghanistan 1999 745
## 2 Brazil 1999 37737
## 3 China 1999 212258
## 4 Afghanistan 2000 2666
## 5 Brazil 2000 80488
## 6 China 2000 213766
gather()
Exampleyear
and cases
. gather()
Exampletable4 %>%
gather("year", "cases", "1999":"2000")
table4 %>%
gather("year", "cases", -1)
population2
from last example:population 2 <- population %>%
spread(year, population)
year
and population
. In the end your data frame should look like:
## # A tibble: 2 x 3
## country year population
## <chr> <int> <int>
## 1 Afghanistan 1995 17586073
## 2 Afghanistan 1996 18415307
dplyr
Packagedplyr
Packagedplyr
package allows us to further work with our data. dplyr
Functionalitydplyr
we have five basic verbs that we will learn to work with:
filter()
select()
arrange()
mutate()
summarize()
filter()
Functionfilter()
function chooses rows that meet a specific criteria. dplyr
. library(dplyr)
gapminder[gapminder$country=="Kenya" & gapminder$year==2002, ]
## # A tibble: 1 x 6
## country continent year lifeExp pop gdpPercap
## <fct> <fct> <int> <dbl> <int> <dbl>
## 1 Kenya Africa 2002 51.0 31386842 1288.
gapminder
and we only want to keep the rows of data there country=="Kenya
and year==2002
. filter()
function to do this in a much easier to read format:filter()
Functionfilter(.data, ...)
where
.data
is a tibble....
is a set of arguments the data you want returned needs to meet. gapminder %>%
filter(country=="Kenya", year==2002)
Finally we could also only do one filtering at a time and chain it:
gapminder %>%
filter(country=="Kenya") %>%
filter(year==2002)
filter()
supports the use of multiple conditions where we can use Boolean. gapminder %>% filter(lifeExp>=49 & lifeExp<60)
## # A tibble: 373 x 6
## country continent year lifeExp pop gdpPercap
## <fct> <fct> <int> <dbl> <int> <dbl>
## 1 Albania Europe 1952 55.2 1282697 1601.
## 2 Albania Europe 1957 59.3 1476505 1942.
## 3 Algeria Africa 1967 51.4 12760499 3247.
## 4 Algeria Africa 1972 54.5 14760787 4183.
## 5 Algeria Africa 1977 58.0 17152804 4910.
## 6 Bahrain Asia 1952 50.9 120447 9867.
## 7 Bahrain Asia 1957 53.8 138655 11636.
## 8 Bahrain Asia 1962 56.9 171863 12753.
## 9 Bahrain Asia 1967 59.9 202182 14805.
## 10 Bangladesh Asia 1982 50.0 93074406 677.
## # ... with 363 more rows
filter()
function to remove missing data for us. is.
foo()
where foo represents a data type. gapminder %>% filter(!is.na(lifeExp))
Using the filter()
function and chaining:
Your end result should be:
## # A tibble: 24 x 6
## country continent year lifeExp pop gdpPercap
## <fct> <fct> <int> <dbl> <int> <dbl>
## 1 Rwanda Africa 1952 40 2534927 493.
## 2 Rwanda Africa 1957 41.5 2822082 540.
## 3 Rwanda Africa 1962 43 3051242 597.
## 4 Rwanda Africa 1967 44.1 3451079 511.
## 5 Rwanda Africa 1972 44.6 3992121 591.
## 6 Rwanda Africa 1977 45 4657072 670.
## 7 Rwanda Africa 1982 46.2 5507565 882.
## 8 Rwanda Africa 1987 44.0 6349365 848.
## 9 Rwanda Africa 1992 23.6 7290203 737.
## 10 Rwanda Africa 1997 36.1 7212583 590.
## # ... with 14 more rows
select()
Functionselect()
function chooses columns that we specify. dplyr
. dplyr
functions instead.gapminder[, c("country", "lifeExp", "year")]
select()
Functionselect(.data, ...)
where
.data
is a tibble....
are the columns that you wish to have in bare (no quotations)We could then do the following
gapminder %>%
select(country, lifeExp, year)
# A tibble: 1,704 x 3
country lifeExp year
<fctr> <dbl> <int>
1 Afghanistan 28.801 1952
2 Afghanistan 30.332 1957
3 Afghanistan 31.997 1962
4 Afghanistan 34.020 1967
5 Afghanistan 36.088 1972
6 Afghanistan 38.438 1977
7 Afghanistan 39.854 1982
8 Afghanistan 40.822 1987
9 Afghanistan 41.674 1992
10 Afghanistan 41.763 1997
# ... with 1,694 more rows
select()
function will also remove columns. gdpPercap
and pop
of the countries:gapminder %>%
select(-gdpPercap,-pop)
We also could use a vector for this:
cols <- c("gdpPercap", "pop")
gapminder %>%
select(-one_of(cols))
gapminder %>%
select(-matches("co"))
## # A tibble: 1,704 x 4
## year lifeExp pop gdpPercap
## <int> <dbl> <int> <dbl>
## 1 1952 28.8 8425333 779.
## 2 1957 30.3 9240934 821.
## 3 1962 32.0 10267083 853.
## 4 1967 34.0 11537966 836.
## 5 1972 36.1 13079460 740.
## 6 1977 38.4 14880372 786.
## 7 1982 39.9 12881816 978.
## 8 1987 40.8 13867957 852.
## 9 1992 41.7 16317921 649.
## 10 1997 41.8 22227415 635.
## # ... with 1,694 more rows
unique()
command. gapminder %>%
select(country) %>%
unique()
gapminder
.
pop
column.Your answer should look like:
## # A tibble: 1,704 x 1
## gdpPercap
## <dbl>
## 1 779.
## 2 821.
## 3 853.
## 4 836.
## 5 740.
## 6 786.
## 7 978.
## 8 852.
## 9 649.
## 10 635.
## # ... with 1,694 more rows
arrange()
function. library(gapminder)
library(tidyverse)
gapminder[order(gapminder$lifeExp), c("country", "year", "lifeExp")]
arrange()
FunctionWe could do this in an easy manner using the arrange()
function:
arrange(.data, ...)
Where
.data
is a data frame of interest....
are the variables you wish to sort by. gapminder %>%
select(country,year, lifeExp) %>%
arrange(lifeExp)
## # A tibble: 1,704 x 3
## country year lifeExp
## <fct> <int> <dbl>
## 1 Rwanda 1992 23.6
## 2 Afghanistan 1952 28.8
## 3 Gambia 1952 30
## 4 Angola 1952 30.0
## 5 Sierra Leone 1952 30.3
## 6 Afghanistan 1957 30.3
## 7 Cambodia 1977 31.2
## 8 Mozambique 1952 31.3
## 9 Sierra Leone 1957 31.6
## 10 Burkina Faso 1952 32.0
## # ... with 1,694 more rows
arrange()
we first use select()
to pick the only columns that we want and then we arrange by the lifeExp
. desc()
function:gapminder %>%
select(country,year, lifeExp) %>%
arrange(desc(lifeExp))
gapminder %>%
group_by(year) %>%
top_n(3, lifeExp) %>%
arrange(desc(lifeExp))
group_by()
is a way to group data. This way we perform operations on a group. So top 3 life expectancy are grouped by year. top_n()
takes a tibble and returns a specific number of rows based on a chosen value. ## # A tibble: 36 x 6
## # Groups: year [12]
## country continent year lifeExp pop gdpPercap
## <fct> <fct> <int> <dbl> <int> <dbl>
## 1 Japan Asia 2007 82.6 127467972 31656.
## 2 Hong Kong, China Asia 2007 82.2 6980412 39725.
## 3 Japan Asia 2002 82 127065841 28605.
## 4 Iceland Europe 2007 81.8 301931 36181.
## 5 Hong Kong, China Asia 2002 81.5 6762476 30209.
## 6 Japan Asia 1997 80.7 125956499 28817.
## 7 Switzerland Europe 2002 80.6 7361757 34481.
## 8 Hong Kong, China Asia 1997 80 6495918 28378.
## 9 Sweden Europe 1997 79.4 8897619 25267.
## 10 Japan Asia 1992 79.4 124329269 26825.
## # ... with 26 more rows
sample_n()
to pick 1 observation per year Your answer may look like:
gapminder %>%
group_by(year) %>%
sample_n(1) %>%
arrange(desc(lifeExp))
summarise()
function.