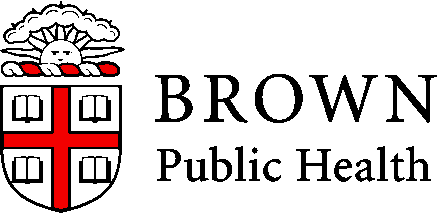
Adam J Sullivan
Assistant Professor of Biostatistics
Brown University
country
.lifeExp
.head(with(gapminder, tapply(lifeExp, country, mean, na.rm=TRUE)))
head(aggregate(lifeExp ~ country, gapminder, mean))
summarise()
Functionsummarise()
function is:summarise(.data, ...)
.data
is the tibble of interest....
is a list of name paired summary functionsmean()
median
var()
sd()
min()
avg_lifeExp
.gapminder %>%
group_by(country) %>%
summarise(avg_lifeExp = mean(lifeExp, na.rm=TRUE))
gapminder %>%
group_by(country) %>%
summarise(avg_lifeExp = mean(lifeExp, na.rm=TRUE))
gapminder %>%
group_by(country) %>%
summarise_each(funs(min(., na.rm=TRUE), max(., na.rm=TRUE)), lifeExp)
## # A tibble: 142 x 3
## country min max
## <fct> <dbl> <dbl>
## 1 Afghanistan 28.8 43.8
## 2 Albania 55.2 76.4
## 3 Algeria 43.1 72.3
## 4 Angola 30.0 42.7
## 5 Argentina 62.5 75.3
## 6 Australia 69.1 81.2
## 7 Austria 66.8 79.8
## 8 Bahrain 50.9 75.6
## 9 Bangladesh 37.5 64.1
## 10 Belgium 68 79.4
## # ... with 132 more rows
n()
counts the number of rows in a groupYour answer should look like:
## # A tibble: 60 x 3
## # Groups: continent [5]
## continent year lifeExp_count
## <fct> <int> <int>
## 1 Africa 1952 52
## 2 Africa 1957 52
## 3 Africa 1962 52
## 4 Africa 1967 52
## 5 Africa 1972 52
## 6 Africa 1977 52
## 7 Africa 1982 52
## 8 Africa 1987 52
## 9 Africa 1992 52
## 10 Africa 1997 52
## # ... with 50 more rows
We could also have used what is called the tally()
function:
gapminder %>%
group_by(country, year) %>%
tally(sort = TRUE)
## # A tibble: 1,704 x 3
## # Groups: country [142]
## country year n
## <fct> <int> <int>
## 1 Afghanistan 1952 1
## 2 Afghanistan 1957 1
## 3 Afghanistan 1962 1
## 4 Afghanistan 1967 1
## 5 Afghanistan 1972 1
## 6 Afghanistan 1977 1
## 7 Afghanistan 1982 1
## 8 Afghanistan 1987 1
## 9 Afghanistan 1992 1
## 10 Afghanistan 1997 1
## # ... with 1,694 more rows
tidyverse
we can add new variables in multiple ways
mutate()
transmute()
mutate()
we have
mutate(.data, ...)
.data
is your tibble of interest....
is the name paired with an expressiontransmute()
we have:
transmute(.data, ...)
.data
is your tibble of interest....
is the name paired with an expressionmutate()
and transmute()
mutate()
and transmutate
and that is what it keeps in your data.
mutate()
transmute()
\[\text{gdp} = gdpPercap\times pop\]
mutate()
:gapminder %>%
select(country, gdpPercap, pop) %>%
mutate(gdp = gdpPercap*pop)
## # A tibble: 1,704 x 4
## country gdpPercap pop gdp
## <fct> <dbl> <int> <dbl>
## 1 Afghanistan 779. 8425333 6567086330.
## 2 Afghanistan 821. 9240934 7585448670.
## 3 Afghanistan 853. 10267083 8758855797.
## 4 Afghanistan 836. 11537966 9648014150.
## 5 Afghanistan 740. 13079460 9678553274.
## 6 Afghanistan 786. 14880372 11697659231.
## 7 Afghanistan 978. 12881816 12598563401.
## 8 Afghanistan 852. 13867957 11820990309.
## 9 Afghanistan 649. 16317921 10595901589.
## 10 Afghanistan 635. 22227415 14121995875.
## # ... with 1,694 more rows
gapminder %>%
select(country, gdpPercap, pop) %>%
transmute(gdp = gdpPercap*pop)
## # A tibble: 1,704 x 1
## gdp
## <dbl>
## 1 6567086330.
## 2 7585448670.
## 3 8758855797.
## 4 9648014150.
## 5 9678553274.
## 6 11697659231.
## 7 12598563401.
## 8 11820990309.
## 9 10595901589.
## 10 14121995875.
## # ... with 1,694 more rows
tally()
and count()
. tally()
in this manner: gapminder %>%
group_by(year) %>%
tally()
count()
gapminder %>%
count(year)
*Notice: count()
allowed for month to be called inside of it, removing the need for the group_by()
function.
tally()
and count()
have an argument called sort()
. tally()
this would be:gapminder %>% group_by(year) %>% tally(sort=TRUE)
tally()
## # A tibble: 12 x 2
## year n
## <int> <int>
## 1 1952 142
## 2 1957 142
## 3 1962 142
## 4 1967 142
## 5 1972 142
## 6 1977 142
## 7 1982 142
## 8 1987 142
## 9 1992 142
## 10 1997 142
## 11 2002 142
## 12 2007 142
summarise()
function, tally()
function or the count()
function:gapminder %>%
group_by(continent, year) %>%
summarise(total_gdp = sum(gdp))
## Error in summarise_impl(.data, dots): Evaluation error: object 'gdp' not found.
gapminder <- gapminder %>%
mutate(gdp=gdpPercap*pop)
## # A tibble: 5 x 2
## continent total_gdp
## <fct> <dbl>
## 1 Africa 1.30e13
## 2 Americas 1.14e14
## 3 Asia 9.00e13
## 4 Europe 9.70e13
## 5 Oceania 4.52e12
tally()
we could do:gapminder %>%
group_by(continent) %>%
tally(wt = gdp)
Note: in tally()
the wt
stands for weight and allows you to weight the sum based on the gdp.
count()
function we also use wt
:gapminder %>% count(continent, wt = gdp)
## # A tibble: 5 x 2
## continent n
## <fct> <dbl>
## 1 Africa 1.30e13
## 2 Americas 1.14e14
## 3 Asia 9.00e13
## 4 Europe 9.70e13
## 5 Oceania 4.52e12
group_size()
is a function that returns counts of group. n_groups()
returns the number of groupsgroup_size()
:gapminder %>%
group_by(continent) %>%
group_size()
## [1] 624 300 396 360 24
n_groups()
function:gapminder %>%
group_by(year) %>%
n_groups()
## [1] 12