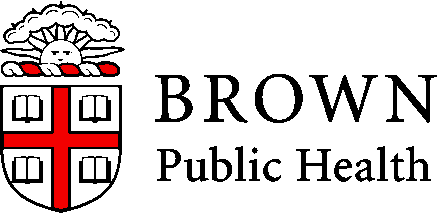
Adam J Sullivan
Assistant Professor of Biostatistics
Brown University
ggplot(comic_characters, aes(year, appearances)) +
geom_point() +
geom_smooth(method="lm") +
xlab("Year") +
ylab("Appearances")
model <- lm(appearances~year, data=comic_characters)
tidy(model, conf.int=TRUE)[,-c(3:4)]
glance(model)
## # A tibble: 1 x 11
## r.squared adj.r.squared sigma statistic p.value df logLik AIC
## <dbl> <dbl> <dbl> <dbl> <dbl> <int> <dbl> <dbl>
## 1 0.0146 0.0145 93.8 313. 1.74e-69 2 -1.26e5 2.52e5
## # ... with 3 more variables: BIC <dbl>, deviance <dbl>, df.residual <int>
## # A tibble: 1 x 11
## r.squared adj.r.squared sigma statistic p.value df logLik AIC
## <dbl> <dbl> <dbl> <dbl> <dbl> <int> <dbl> <dbl>
## 1 0.0146 0.0145 93.8 313. 1.74e-69 2 -1.26e5 2.52e5
## # ... with 3 more variables: BIC <dbl>, deviance <dbl>, df.residual <int>
mod3 <- lm(appearances~publisher + year, data=comic_characters)
tidy3 <- tidy(mod3, conf.int=T)[,-c(3:4)]
tidy3
## # A tibble: 3 x 5
## term estimate p.value conf.low conf.high
## <chr> <dbl> <dbl> <dbl> <dbl>
## 1 (Intercept) 1265. 9.81e-78 1133. 1398.
## 2 publisherMarvel -9.54 1.24e-11 -12.3 -6.78
## 3 year -0.624 5.93e-75 -0.690 -0.557
Race | Prostatectomy | No Prostatectomy | |
---|---|---|---|
University Hospital | White | 54 | 37 |
Black | 7 | 5 | |
VA Hospital | White | 11 | 29 |
Black | 22 | 57 |
You can load this data into R with the code below:
phil_disp <- read.table("https://drive.google.com/uc?export=download&id=0B8CsRLdwqzbzOXlIRl9VcjNJRFU", header=TRUE, sep=",")
This dataset contains the following variables:
Variable | Description |
---|---|
hospital | 0 - University Hospital |
1 - VA Hospital | |
race | 0 - White |
1 - Black | |
surgery | 0 - No prostatectomy |
1 - Had Prostatectomy | |
number | Count of people in Category |
library(broom)
prost_race <- glm(surgery ~ race, weight=number, data= phil_disp,
family="binomial")
tidy(prost_race, exponentiate=T, conf.int=T)[,-c(3:4)]
## # A tibble: 2 x 5
## term estimate p.value conf.low conf.high
## <chr> <dbl> <dbl> <dbl> <dbl>
## 1 (Intercept) 0.985 0.930 0.699 1.39
## 2 race 0.475 0.00895 0.269 0.825
prost_hosp <- glm(surgery ~ hospital, weight=number, data= phil_disp,
family="binomial")
tidy(prost_hosp, exponentiate =T, conf.int=T)[,-c(3:4)]
## # A tibble: 2 x 5
## term estimate p.value conf.low conf.high
## <chr> <dbl> <dbl> <dbl> <dbl>
## 1 (Intercept) 1.45 0.0627 0.984 2.16
## 2 hospital 0.264 0.00000341 0.149 0.460
prost <- glm(surgery ~ hospital + race, weight=number, data= phil_disp,
family="binomial")
tidy(prost, exponentiate=T, conf.int=T)[,-c(3:4)]
## # A tibble: 3 x 5
## term estimate p.value conf.low conf.high
## <chr> <dbl> <dbl> <dbl> <dbl>
## 1 (Intercept) 1.45 0.0682 0.976 2.18
## 2 hospital 0.264 0.000124 0.131 0.515
## 3 race 0.998 0.996 0.501 2.04
library(broom)
library(fivethirtyeight)
mod3 <- lm(appearances~publisher + year, data=comic_characters)
tidy3 <- tidy(mod3, conf.int=T)[,-c(3:4)]
tidy3
## # A tibble: 3 x 5
## term estimate p.value conf.low conf.high
## <chr> <dbl> <dbl> <dbl> <dbl>
## 1 (Intercept) 1265. 9.81e-78 1133. 1398.
## 2 publisherMarvel -9.54 1.24e-11 -12.3 -6.78
## 3 year -0.624 5.93e-75 -0.690 -0.557
Data | Fields |
---|---|
datetime | hourly date + timestamp |
season | 1 = spring, 2 = summer, 3 = fall, 4 = winter |
holiday | whether the day is considered a holiday |
workingday | whether the day is neither a weekend nor holiday |
Data | Fields |
---|---|
weather | 1: Clear, Few clouds, Partly cloudy, Partly cloudy |
2: Mist + Cloudy, Mist + Broken clouds, Mist + Few clouds, Mist | |
3: Light Snow, Light Rain + Thunderstorm + Scattered clouds, Light Rain + Scattered clouds | |
4: Heavy Rain + Ice Pallets + Thunderstorm + Mist, Snow + Fog | |
temp | temperature in Celsius |
Data | Fields |
---|---|
atemp | "feels like" temperature in Celsius |
humidity | relative humidity |
windspeed | wind speed |
casual | number of non-registered user rentals initiated |
registered | number of registered user rentals initiated |
count | number of total rentals |
library(readr)
library(tidyverse)
bikes <- read_csv("../Notes/Data/bike_sharing.csv") %>%
mutate(season = as.factor(season)) %>%
mutate(weather=as.factor(weather))
mod1 <- lm(count~season, data=bikes)
mod2 <- lm(count~holiday, data=bikes)
mod3 <- lm(count~workingday, data=bikes)
mod4 <- lm(count~weather, data=bikes)
mod5 <- lm(count~temp, data=bikes)
mod6 <- lm(count~atemp, data=bikes)
mod7 <- lm(count~humidity, data=bikes)
mod8 <- lm(count~windspeed, data=bikes)
mod9 <- lm(count~casual, data=bikes)
mod10 <- lm(count~registered, data=bikes)
library(broom)
tidy1 <- tidy( mod1, conf.int=T )[-1, -c(3:4) ]
tidy2 <- tidy(mod2, conf.int=T )[-1, -c(3:4) ]
tidy3 <- tidy(mod3 , conf.int=T)[-1, -c(3:4) ]
tidy4 <- tidy(mod4 , conf.int=T)[-1, -c(3:4) ]
tidy5 <- tidy(mod5, conf.int=T)[-1, -c(3:4) ]
tidy6 <- tidy(mod6 , conf.int=T)[-1, -c(3:4) ]
tidy7 <- tidy(mod7 , conf.int=T)[-1, -c(3:4) ]
tidy8 <- tidy(mod8 , conf.int=T)[-1, -c(3:4) ]
tidy9 <- tidy(mod9, conf.int=T)[-1, -c(3:4) ]
tidy10 <- tidy(mod10, conf.int=T)[-1, -c(3:4) ]
bind_rows(tidy1, tidy2, tidy3, tidy4, tidy5, tidy6, tidy7, tidy8, tidy9, tidy10)
## # A tibble: 14 x 5
## term estimate p.value conf.low conf.high
## <chr> <dbl> <dbl> <dbl> <dbl>
## 1 season2 98.9 9.76e- 94 89.6 108.
## 2 season3 118. 1.06e-131 109. 127.
## 3 season4 82.6 2.13e- 66 73.3 92.0
## 4 holiday -5.86 5.74e- 1 -26.3 14.6
## 5 workingday 4.51 2.26e- 1 -2.80 11.8
## 6 weather2 -26.3 4.32e- 11 -34.1 -18.5
## 7 weather3 -86.4 3.29e- 40 -99.1 -73.7
## 8 weather4 -41.2 8.18e- 1 -393. 311.
## 9 temp 9.17 0. 8.77 9.57
## 10 atemp 8.33 0. 7.96 8.70
## 11 humidity -2.99 2.92e-253 -3.15 -2.82
## 12 windspeed 2.25 2.90e- 26 1.83 2.66
## 13 casual 2.50 0. 2.45 2.55
## 14 registered 1.16 0. 1.16 1.17
mod.final <- lm(count~season+weather+humidity+windspeed, data=bikes)
tidy(mod.final)[-1,-c(3:4)]
glance(mod.final)
## # A tibble: 8 x 3
## term estimate p.value
## <chr> <dbl> <dbl>
## 1 season2 116. 1.40e-145
## 2 season3 148. 7.52e-227
## 3 season4 118. 1.74e-147
## 4 weather2 20.0 1.38e- 7
## 5 weather3 0.124 9.84e- 1
## 6 weather4 162. 3.19e- 1
## 7 humidity -3.49 3.86e-273
## 8 windspeed 0.633 2.05e- 3
## # A tibble: 1 x 11
## r.squared adj.r.squared sigma statistic p.value df logLik AIC
## <dbl> <dbl> <dbl> <dbl> <dbl> <int> <dbl> <dbl>
## 1 0.195 0.194 163. 329. 0 9 -70865. 1.42e5
## # ... with 3 more variables: BIC <dbl>, deviance <dbl>, df.residual <int>