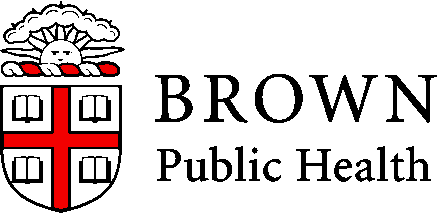
Adam J Sullivan
Assistant Professor of Biostatistics
Brown University
Variable Name | Description |
---|---|
Subject | subject number in the study |
Metabol | first–pass metabolism of alcohol in the stomach (in mmol/liter-hour) |
Gastric | gastric alcohol dehydrogenase activity in the stomach (in mumol/min/g of tissue) |
Sex | sex of the subject |
Alcohol | whether the subject is alcoholic or not |
## # A tibble: 2 x 5
## term estimate p.value conf.low conf.high
## <chr> <dbl> <dbl> <dbl> <dbl>
## 1 (Intercept) -1.83 0.00428 -3.03 -0.620
## 2 Gastric 2.28 0.00000000527 1.70 2.86
## # A tibble: 3 x 5
## term estimate p.value conf.low conf.high
## <chr> <dbl> <dbl> <dbl> <dbl>
## 1 (Intercept) -1.95 0.000796 -3.01 -0.884
## 2 Gastric 1.97 0.0000000424 1.42 2.51
## 3 SexMale 1.62 0.00365 0.572 2.66
## # A tibble: 4 x 5
## term estimate p.value conf.low conf.high
## <chr> <dbl> <dbl> <dbl> <dbl>
## 1 (Intercept) -0.197 0.808 -1.84 1.45
## 2 Gastric 0.837 0.0947 -0.154 1.83
## 3 SexMale -0.988 0.365 -3.19 1.21
## 4 Gastric:SexMale 1.51 0.0118 0.362 2.65
## # A tibble: 3 x 5
## term estimate p.value conf.low conf.high
## <chr> <dbl> <dbl> <dbl> <dbl>
## 1 (Intercept) -0.750 0.168 -1.84 0.336
## 2 Gastric 1.15 0.00237 0.443 1.85
## 3 Gastric:SexMale 1.04 0.000166 0.549 1.54
\[Metabolism = \beta_0 + \beta_1 Gastric + \beta_2 Gastric*Male\]
gpa_data %>%
summarise(`0%`=quantile(iq, probs=0),
`33%`=quantile(iq, probs=0.33),
`66%`=quantile(iq, probs=0.66),
`100%`=quantile(iq,probs=1))
## 0% 33% 66% 100%
## 1 53.44964 92.72525 106.0709 136.3098
gpa_data <-gpa_data %>%
mutate(iq_fact = cut(iq, 3, labels =c('low', 'medium', 'high')))
mod <- lm(data=gpa_data, gpa~work_ethic*iq)
tidy(mod, conf.int = T)[,-c(3:4)]
## # A tibble: 4 x 5
## term estimate p.value conf.low conf.high
## <chr> <dbl> <dbl> <dbl> <dbl>
## 1 (Intercept) -0.857 1.85e-166 -0.880 -0.833
## 2 work_ethic -0.000492 9.05e- 1 -0.00857 0.00758
## 3 iq 0.00129 1.28e- 22 0.00106 0.00153
## 4 work_ethic:iq 0.00894 5.35e-284 0.00886 0.00902
anscombe
## x1 x2 x3 x4 y1 y2 y3 y4
## 1 10 10 10 8 8.04 9.14 7.46 6.58
## 2 8 8 8 8 6.95 8.14 6.77 5.76
## 3 13 13 13 8 7.58 8.74 12.74 7.71
## 4 9 9 9 8 8.81 8.77 7.11 8.84
## 5 11 11 11 8 8.33 9.26 7.81 8.47
## 6 14 14 14 8 9.96 8.10 8.84 7.04
## 7 6 6 6 8 7.24 6.13 6.08 5.25
## 8 4 4 4 19 4.26 3.10 5.39 12.50
## 9 12 12 12 8 10.84 9.13 8.15 5.56
## 10 7 7 7 8 4.82 7.26 6.42 7.91
## 11 5 5 5 8 5.68 4.74 5.73 6.89
mod1 <- lm(y1 ~ x1, data=anscombe)
mod2 <- lm(y2 ~ x2, data=anscombe)
mod3 <- lm(y3 ~ x3, data=anscombe)
mod4 <- lm(y4 ~ x4, data=anscombe)
library(broom)
library(dplyr)
tidy1 <- tidy(mod1, conf.int = T)
tidy2 <- tidy(mod2, conf.int = T)
tidy3 <- tidy(mod3, conf.int = T)
tidy4 <- tidy(mod4, conf.int = T)
knitr::kable(bind_rows(tidy1,tidy2, tidy3, tidy4)[,-c(3,4)])
term | estimate | p.value | conf.low | conf.high |
---|---|---|---|---|
(Intercept) | 3.0000909 | 0.0257341 | 0.4557369 | 5.5444449 |
x1 | 0.5000909 | 0.0021696 | 0.2333701 | 0.7668117 |
(Intercept) | 3.0009091 | 0.0257589 | 0.4552982 | 5.5465200 |
x2 | 0.5000000 | 0.0021788 | 0.2331475 | 0.7668525 |
(Intercept) | 3.0024545 | 0.0256191 | 0.4587013 | 5.5462078 |
x3 | 0.4997273 | 0.0021763 | 0.2330695 | 0.7663851 |
(Intercept) | 3.0017273 | 0.0255904 | 0.4592412 | 5.5442134 |
x4 | 0.4999091 | 0.0021646 | 0.2333841 | 0.7664341 |
glance1 <- glance(mod1)
glance2 <- glance(mod2)
glance3 <- glance(mod3)
glance4 <- glance(mod4)
knitr::kable(bind_rows(glance1,glance2, glance3, glance4))
r.squared | adj.r.squared | sigma | statistic | p.value | df | logLik | AIC | BIC | deviance | df.residual |
---|---|---|---|---|---|---|---|---|---|---|
0.6665425 | 0.6294916 | 1.236603 | 17.98994 | 0.0021696 | 2 | -16.84069 | 39.68137 | 40.87506 | 13.76269 | 9 |
0.6662420 | 0.6291578 | 1.237214 | 17.96565 | 0.0021788 | 2 | -16.84612 | 39.69224 | 40.88593 | 13.77629 | 9 |
0.6663240 | 0.6292489 | 1.236311 | 17.97228 | 0.0021763 | 2 | -16.83809 | 39.67618 | 40.86986 | 13.75619 | 9 |
0.6667073 | 0.6296747 | 1.235696 | 18.00329 | 0.0021646 | 2 | -16.83261 | 39.66522 | 40.85890 | 13.74249 | 9 |
library(ggplot2)
library(gridExtra)
p1 <- ggplot(anscombe, aes(x1,y1)) + geom_point() + geom_smooth(method="lm", se=FALSE)+theme_bw()
p2 <- ggplot(anscombe, aes(x2,y2)) + geom_point() + geom_smooth(method="lm", se=FALSE)+theme_bw()
p3 <- ggplot(anscombe, aes(x3,y3)) + geom_point() + geom_smooth(method="lm", se=FALSE)+theme_bw()
p4 <- ggplot(anscombe, aes(x4,y4)) + geom_point() + geom_smooth(method="lm", se=FALSE)+theme_bw()
grid.arrange(p1,p2,p3, p4, ncol=2)
plot(mod1,1)
plot(mod2,1)
plot(mod3,1)
plot(mod4,1)
anscombe$x2sq <- anscombe$x2^2
mod2a <- lm(y2 ~ x2 + x2sq, data=anscombe)
tidy(mod2a, conf.int=T)[,-c(3,4)]
glance(mod2a)
plot(mod2a,1)
term | estimate | p.value | conf.low | conf.high |
---|---|---|---|---|
(Intercept) | -5.9957343 | 0 | -6.005719 | -5.9857494 |
x2 | 2.7808392 | 0 | 2.778441 | 2.7832375 |
x2sq | -0.1267133 | 0 | -0.126845 | -0.1265816 |
r.squared | adj.r.squared | sigma | statistic | p.value | df | logLik | AIC | BIC | deviance | df.residual |
---|---|---|---|---|---|---|---|---|---|---|
0.9999995 | 0.9999993 | 0.0016725 | 7378133 | 0 | 3 | 56.47107 | -104.9421 | -103.3506 | 2.24e-05 | 8 |
When fitting a regression model we will take these steps to verify the validity of the model:
\[E[Y|x] = \beta_0 + \beta_1 x + \beta_2 x^2\]
x2
has been transformed to a square term, the \(beta\) values are still linear. plot(mod2,1)
plot(mod2a, 2)
mean(mod2a$residuals)
## [1] 0