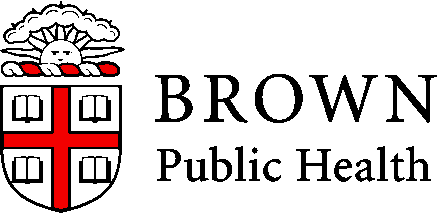
Adam J Sullivan
Assistant Professor of Biostatistics
Brown University
## response predictor1 predictor2
## response 1.000 0.8 0.202
## predictor1 0.800 1.0 0.000
## predictor2 0.202 0.0 1.000
term | estimate | p.value | conf.low | conf.high |
---|---|---|---|---|
predictor1 | 0.8003296 | 0 | 0.7966066 | 0.8040526 |
predictor2 | 0.2016739 | 0 | 0.1956074 | 0.2077403 |
predictor1 | 0.8004000 | 0 | 0.7968944 | 0.8039057 |
predictor2 | 0.2019523 | 0 | 0.1984514 | 0.2054533 |
predictor2 | 0.2019523 | 0 | 0.1984514 | 0.2054533 |
predictor1 | 0.8004000 | 0 | 0.7968944 | 0.8039057 |
r.squared | adj.r.squared | sigma | statistic | p.value |
---|---|---|---|---|
0.6396798 | 0.6396762 | 0.6008585 | 177527.401 | 0 |
0.0407269 | 0.0407173 | 0.9803905 | 4245.513 | 0 |
0.6805193 | 0.6805129 | 0.5657864 | 106500.764 | 0 |
0.6805193 | 0.6805129 | 0.5657864 | 106500.764 | 0 |
term | df | sumsq | meansq |
---|---|---|---|
predictor1 | 1 | 64092.895 | 64092.895 |
predictor2 | 1 | 4080.641 | 4080.641 |
predictor1 | 1 | 64092.895 | 64092.895 |
predictor2 | 1 | 4091.918 | 4091.918 |
predictor2 | 1 | 4080.641 | 4080.641 |
predictor1 | 1 | 64104.172 | 64104.172 |
## response predictor1 predictor2
## response 1.000 0.846 0.188
## predictor1 0.846 1.000 0.639
## predictor2 0.188 0.639 1.000
term | estimate | p.value | conf.low | conf.high |
---|---|---|---|---|
predictor1 | 0.9289612 | 0.0000000 | 0.8117562 | 1.0461662 |
predictor2 | 0.2349541 | 0.0606592 | -0.0107236 | 0.4806318 |
predictor1 | 1.3475785 | 0.0000000 | 1.2697073 | 1.4254497 |
predictor2 | -0.7444484 | 0.0000000 | -0.8329782 | -0.6559187 |
predictor2 | -0.7444484 | 0.0000000 | -0.8329782 | -0.6559187 |
predictor1 | 1.3475785 | 0.0000000 | 1.2697073 | 1.4254497 |
r.squared | adj.r.squared | sigma | statistic | p.value |
---|---|---|---|---|
0.7162667 | 0.7133715 | 0.5868151 | 247.394788 | 0.0000000 |
0.0354504 | 0.0256081 | 1.0819531 | 3.601826 | 0.0606592 |
0.9267136 | 0.9252025 | 0.2997678 | 613.287170 | 0.0000000 |
0.9267136 | 0.9252025 | 0.2997678 | 613.287170 | 0.0000000 |
term | df | sumsq | meansq |
---|---|---|---|
predictor1 | 1 | 85.190885 | 85.190885 |
predictor2 | 1 | 4.216378 | 4.216378 |
predictor1 | 1 | 85.190885 | 85.190885 |
predictor2 | 1 | 25.030002 | 25.030002 |
predictor2 | 1 | 4.216378 | 4.216378 |
predictor1 | 1 | 106.004508 | 106.004508 |
\[y_i= \beta_0 + \beta_kx_{ik} + \varepsilon_i\]
\[y_i= \beta_0 + \beta_1x_{i1} + \cdots + \beta_kx_{ik} + \cdots \beta_px_{ip} \varepsilon_i\]
\[\dfrac{Var(b_k)}{Var(b_k)_{min}} =\dfrac{1}{1-R^2_k} \]
library(car)
vif1 <- vif(mod3)
vif2 <- vif(mod4)
knitr::kable(bind_rows(vif1,vif2))
predictor1 | predictor2 |
---|---|
1 | 1 |
1 | 1 |
predictor1 | predictor2 |
---|---|
1.691149 | 1.691149 |
1.691149 | 1.691149 |
We use \[\eta_i = \beta_0 + \beta_1x_{i1} + \beta_2x_{i2} + \cdots + \beta_px_{ip} = \sum_{j=1}^p \beta_jx_{ij}\]
where:
Some common link functions are
Random Component | Link Function | Outcome | Explanatory | Model |
---|---|---|---|---|
Normal | Identity | Continuous | Factor | ANOVA |
Normal | Identity | Continuous | Continuous | Regression |
Binomial | Logit | Binary | Mixed | Logistic Regression |
Multinomial | Generalized logit | Binary | Mixed | Multinomial Regression |
Poisson | Log | Count | Mixed | Poisson Regression |
\[ Y = \begin{cases} 1 & \text{if sucess}\\ 0 & \text{if failure} \end{cases} \]
\[E(Y) = np\] \[Var(Y)= np(1-p)\]
\[ \begin{aligned} p_i &= \dfrac{\exp\left(\beta_0 + \beta_1x_i\right)}{1+\exp\left(\beta_0 + \beta_1x_i\right)}\\ p_i\left(1+\exp\left(\beta_0 + \beta_1x_i\right)\right)&=\exp\left(\beta_0 + \beta_1x_i\right)\\ p_i &= \exp\left(\beta_0 + \beta_1x_i\right)\left(1-p_i\right)\\ \log\left(\dfrac{p_i}{1-p_i}\right) &= \beta_0 + \beta_1x_i\\ logit\left(p_i\right) &= \beta_0 + \beta_1x_i \end{aligned} \]
Then if we consider the logit:
\[ \begin{aligned} \text{If } p= 0 & \text{then } \log\left(\dfrac{p}{1-p}\right)=-\infty\\ \text{If } p= \tfrac{1}{2} & \text{then } \log\left(\dfrac{p}{1-p}\right)=0\\ \text{If } p= 1 & \text{then } \log\left(\dfrac{p}{1-p}\right)=\infty \end{aligned} \]
\[ \begin{aligned} \eta &= \beta_0 + \beta_1x_{i1} + \cdots + \beta_px_{ip} \\ g(E(y_i)) &= \beta_0 + \beta_1x_{i1} + \cdots + \beta_px_{ip} \\ g(p_i) &= logit\left(p_i\right) \end{aligned} \]
\[ \begin{aligned} \Pr(Y_i=0|x_i) &= 1- \Pr(Y_i=1|x_i)\\ &= 1 - \dfrac{\exp\left(\beta_0 + \beta_1x_i\right)}{1+ \exp\left(\beta_0+\beta_1x_i\right)}\\ &= \dfrac{1}{1+ \exp\left(\beta_0+\beta_1x_i\right)} \end{aligned} \]