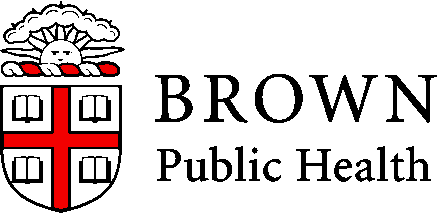
Adam J Sullivan
Assistant Professor of Biostatistics
Brown University
chd69 | arcus | n |
---|---|---|
0 | 0 | 2058 |
0 | 1 | 839 |
1 | 0 | 153 |
1 | 1 | 102 |
1 | NA | 2 |
Absent | Present | |
---|---|---|
no | 2058 | 839 |
yes | 153 | 102 |
We can then find the following:
This leads to \[ \begin{aligned} \log\left(\dfrac{p_1}{1-p_1}\right) = \beta_0+\beta_1 &\Rightarrow p_1 = \dfrac{\exp\left(\beta_0+\beta_1\right)}{1+\exp\left(\beta_0+\beta_1\right)} \\ \log\left(\dfrac{p_0}{1-p_0}\right) = \beta_0 & \Rightarrow p_0 = \dfrac{\exp\left(\beta_0\right)}{1+\exp\left(\beta_0\right)}\\ \end{aligned} \]
\[ \begin{aligned} \beta_0 &= \log\left(\dfrac{p_0}{1-p_0}\right)\\ \beta_1 &= \log\left(\dfrac{p_1}{1-p_1}\right) - \beta_0\\ &= \log\left(\dfrac{p_1}{1-p_1}\right) - \log\left(\dfrac{p_0}{1-p_0}\right)\\ &= \log\left(\dfrac{\left(\dfrac{p_1}{1-p_1}\right)}{\left(\dfrac{p_0}{1-p_0}\right)}\right)\\ &= \log\left( \dfrac{\text{Odds of CHD in Arcus Present Group}}{\text{Odds of CHD in Arcus Absent Group}}\right)\\ &= \log\left( \text{Odds Ratio}\right) \end{aligned} \]
-This shows us that \(\beta_1\) is the log odds ratio comparing those with Arcus Senilis vs those without it.
\[ \begin{aligned} \beta_1 &= \log\left( \text{Odds Ratio}\right)\\ \text{Odds Ratio} &= \exp\left(\beta_1\right)\\ \end{aligned} \]
fit.bin <- glm(chd69 ~ arcus, data=wcgs, family=binomial(link="logit"))
tidy(fit.bin, exponentiate = TRUE, conf.int=TRUE)[,-c(3:4)]
## # A tibble: 2 x 5
## term estimate p.value conf.low conf.high
## <chr> <dbl> <dbl> <dbl> <dbl>
## 1 (Intercept) 0.0743 3.25e-211 0.0628 0.0873
## 2 arcus 1.64 2.48e- 4 1.25 2.12
When we work with clinical and epidemiological data we tend to focus on multiple predictors. For example:
\[\Pr(Y=1|X_1,\ldots,X_p) = \dfrac{\exp\left( \beta_0 + \beta_1X_1 + \cdots + \beta_pX_p\right)}{1 + \exp\left( \beta_0 + \beta_1X_1 + \cdots + \beta_pX_p\right)} \]
fit.mult <- glm(chd69 ~ age + arcus + chol + sbp, data=wcgs, family=binomial(link="logit"))
tidy(fit.mult, conf.int=TRUE, exponentiate=TRUE)[,-c(3:4)]
term | estimate | p.value | conf.low | conf.high |
---|---|---|---|---|
(Intercept) | 0.0000232 | 0.0000000 | 0.0000048 | 0.0001079 |
age | 1.0586969 | 0.0000024 | 1.0338946 | 1.0841052 |
arcus | 1.2819917 | 0.0793992 | 0.9690163 | 1.6891421 |
chol | 1.0112888 | 0.0000000 | 1.0083450 | 1.0142734 |
sbp | 1.0215975 | 0.0000000 | 1.0137544 | 1.0293837 |
log.odd <-fit.mult$coefficients[1] + fit.mult$coefficients[2]*60 + fit.mult$coefficients[3]*1 +
fit.mult$coefficients[4]*253 + fit.mult$coefficients[5]*136
log.odd
## (Intercept)
## -1.255354
exp(log.odd)/(1 + exp(log.odd))
## (Intercept)
## 0.2217746
Name | Description |
---|---|
age | Age in years at time of Randomization |
asa | 0 - placebo, 1 - aspirin |
bmi | Body Mass Index (kg/\(m^2\)) |
hypert | 1 - Hypertensive at baseline, 0 - Not |
alcohol | 0 - less than monthly, 1 - monthly to less than daily, 2 - daily consumption |
dm | 0 = No diabetes Mellitus, 1 - diabetes Mellitus |
sbp | Systolic BP (mmHg) |
Name | Description |
---|---|
exer | 0 - No regular, 1 - Sweat at least once per week |
csmoke | 0 - Not currently, 1 - < 1 pack per day, 2 - \(\ge\) 1 pack per day |
psmoke | 0 - never smoked, 1 - former < 1 pack per day, 2 - former \(\ge\) 1 pack per day |
pkyrs | Total lifetime packs of cigarettes smoked |
crc | 0 - No colorectal Cancer, 1 - Colorectal cancer |
cayrs | Years to colorectal cancer, or death, or end of follow-up. |
We can load this data into R.
library(tidyverse)
library(haven)
phscrc <- read_dta("phscrc.dta")
phscrc <- phscrc %>%
mutate(age.cat = cut(age, c(40,50,60,70, 90), right=FALSE)) %>%
mutate(alcohol.use = factor(phscrc$alcohol>0, labels=c("no", "yes")))
mytable <- xtabs(~age.cat+alcohol.use+ crc, phscrc)
library(pander)
pandoc.table(mytable)
crc | 0 | 1 | ||
age.cat | alcohol.use | |||
[40,50) | no | 1762 | 3 | |
yes | 4764 | 36 | ||
[50,60) | no | 1385 | 19 | |
yes | 3969 | 61 | ||
[60,70) | no | 749 | 21 | |
yes | 2167 | 73 | ||
[70,90) | no | 278 | 9 | |
yes | 690 | 32 |
We could run the following model: \[CRC = \beta_0 + \beta_1 E + \beta_2 X\]
Where \(E=1\) is the group with people who drink more than monthly and \(X\) is age in years.
\[ \begin{aligned} log&\left(\dfrac{p_{e,x+1}}{1-p_{e,x+1}}\right) - \log\left(\dfrac{p_{e,x}}{1-p_{e,x}}\right)\\ &= (\beta_0 + \beta_1e+ \beta_2(x+1)) - (\beta_0 + \beta_1e+\beta_2x)\\ &= \beta_2\\ \end{aligned} \]
library(tidyverse)
mod1 <- glm(crc ~ alcohol.use+ age, phscrc, family=binomial(link="logit"))
mod2 <- glm(crc ~ alcohol.use*age, phscrc, family=binomial(link="logit"))
phscrc <- phscrc %>%
mutate(age.cent = age - mean(age,na.rm=TRUE))
mod3 <- glm(crc ~ alcohol.use*age.cent, phscrc, family=binomial(link="logit"))
term | estimate | p.value | conf.low | conf.high |
---|---|---|---|---|
(Intercept) | -8.3348992 | 0.0000000 | -9.1304585 | -7.5591081 |
alcohol.useyes | 0.3560274 | 0.0236256 | 0.0560273 | 0.6739932 |
age | 0.0697425 | 0.0000000 | 0.0575685 | 0.0819576 |
term | estimate | p.value | conf.low | conf.high |
---|---|---|---|---|
(Intercept) | -8.5800802 | 0.000000 | -10.2022552 | -7.0380756 |
alcohol.useyes | 0.6722965 | 0.460657 | -1.0882345 | 2.4909110 |
age | 0.0737894 | 0.000000 | 0.0482466 | 0.0995000 |
alcohol.useyes:age | -0.0052401 | 0.723872 | -0.0344218 | 0.0238241 |
term | estimate | p.value | conf.low | conf.high |
---|---|---|---|---|
(Intercept) | -4.6573481 | 0.000000 | -5.0165614 | -4.3396824 |
alcohol.useyes | 0.3937278 | 0.039862 | 0.0325311 | 0.7864981 |
age.cent | 0.0737894 | 0.000000 | 0.0482466 | 0.0995000 |
alcohol.useyes:age.cent | -0.0052401 | 0.723872 | -0.0344218 | 0.0238241 |
\[ \begin{aligned} LR &= 2\log\left(\dfrac{L(\text{Larger Model})}{L(\text{smaller model})}\right)\\ &= 2\log\left(L(\text{Larger Model}\right)- 2\log\left(L(\text{smaller model})\right)\\ \end{aligned} \]
\[H_0: \beta_1 = \beta_2 = \cdots = \beta_p =0\]
null.deviance | df.null | logLik | AIC | BIC | deviance | df.residual |
---|---|---|---|---|---|---|
2609.171 | 16017 | -1240.335 | 2486.67 | 2509.715 | 2480.67 | 16015 |
LR <- summary(mod1)$null.deviance - summary(mod1)$deviance
df <- summary(mod1)$df.null - summary(mod1)$df.residual
1- pchisq(LR,df)
## [1] 0
Many times we have categorical covariates and we need to be able to use them in model building. We can proceed two different ways depending on the data.
If we have covariate \(X\) which is categorical than we treat \(X\) differently depending on
With \(X\) which has \(K\ge2\) categories, we created \(K-1\) variables. For example:
Category | Log Odds | Probability |
---|---|---|
K | \(\beta_0\) | \(p_0 = \dfrac{\exp(\beta_0)}{1+\exp(\beta_0)}\) |
1 | \(\beta_0+\beta_1\) | \(p_0 = \dfrac{\exp(\beta_0+\beta_1)}{1+\exp(\beta_0+\beta_1)}\) |
2 | \(\beta_0+\beta_2\) | \(p_0 = \dfrac{\exp(\beta_0+\beta_2)}{1+\exp(\beta_0+\beta_2)}\) |
\(\vdots\) | \(\vdots\) | \(\vdots\) |
K-1 | \(\beta_0+\beta_{k-1}\) | \(p_0 = \dfrac{\exp(\beta_0+\beta_{k-1})}{1+\exp(\beta_0+\beta_{k-1})}\) |
Alcohol
and its effect of Colorectal Cancer. term | estimate | p.value | conf.low | conf.high |
---|---|---|---|---|
(Intercept) | -4.4182131 | 0.0000000 | -4.6563690 | -4.1931173 |
alcohol | 0.2776848 | 0.0019606 | 0.1023961 | 0.4541972 |
\[ \text{Alcohol} = \begin{cases} 0 & \text{ Less than monthly}\\ 1 & \text{ Monthly to less than Daily}\\ 2 & \text{ Daily or more} \end{cases} \]
This means if
\[
\begin{aligned}
\beta_1 = 0 \Rightarrow p_0=p_1=p_2\\
\beta_1 > 0 \Rightarrow p_0
-We will find that Model 1 is nested within Model 2.
term | estimate | p.value | conf.low | conf.high |
---|---|---|---|---|
(Intercept) | -4.3853864 | 0.0000000 | -4.6716150 | -4.1235215 |
factor(alcohol)1 | 0.2173767 | 0.1928240 | -0.1035724 | 0.5522374 |
factor(alcohol)2 | 0.5436799 | 0.0024019 | 0.1961081 | 0.8999708 |
anova_mod <-anova(mod4, mod5, test="Chisq")
kable(tidy(anova_mod))
Resid..Df | Resid..Dev | df | Deviance | p.value |
---|---|---|---|---|
16016 | 2599.51 | NA | NA | NA |
16015 | 2599.33 | 1 | 0.1807433 | 0.6707353 |