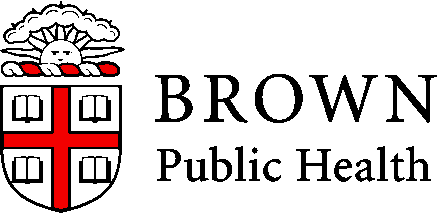
Adam J Sullivan
Assistant Professor of Biostatistics
Brown University
Variable | Description |
---|---|
id Identification Number | |
age Age (years) | |
male Sex | |
1 = Male | |
0 = Female | |
sah | Self Assessed Health |
1 = Excellent | |
2 = Good | |
3 = Fair | |
4= Poor |
Variable | Description |
---|---|
pperf | Physical Performance Scale (0-12) |
pefr | Peak Expiratory Flow Rate (average of 3) |
cogerr | Number of cognitive errors on SPMSQ |
sbp | Systolic Blood Pressure (mmHg) |
mile | Ability to Walk 1 mile |
0 = No | |
1 = Yes | |
digit | Use of digitalis |
0 = No | |
1 = Yes |
Variable | Description |
---|---|
loop | Use of Loop Diuretics |
0 = No | |
1 = Yes | |
untrt | Diagnosed but untreated diabetes |
0 = No | |
1 = Yes |
Variable | Description |
---|---|
trtdb | Treated Diabetes |
0 = No | |
1 = Yes | |
dead | Dead by 1991 |
0 = No | |
1 = Yes | |
time | Follow up (years) |
pperf
and cogerr
sbp
and pefr
untrt
and trtdb
male
, mile
, loop
, digit
and death
library(haven)
sah <- read_dta("sah.dta")
## Start: AIC=1162
## dead ~ sah2 + age + male + pperf + perf + cogerr + sbp + mile +
## digit + loop + untrt + trtdp
##
## Df Deviance AIC
## - age 1 1137 1161
## - digit 1 1137 1161
## - sbp 1 1138 1162
## <none> 1136 1162
## - sah2 1 1139 1163
## - pperf 1 1139 1163
## - perf 1 1140 1164
## - untrt 1 1141 1165
## - mile 1 1144 1168
## - cogerr 1 1145 1169
## - trtdp 1 1146 1170
## - loop 1 1149 1173
## - male 1 1177 1201
##
## Step: AIC=1161
## dead ~ sah2 + male + pperf + perf + cogerr + sbp + mile + digit +
## loop + untrt + trtdp
##
## Df Deviance AIC
## - digit 1 1138 1160
## <none> 1137 1161
## - sbp 1 1139 1161
## - sah2 1 1140 1162
## - pperf 1 1142 1164
## - untrt 1 1142 1164
## - perf 1 1142 1164
## - mile 1 1145 1167
## - trtdp 1 1147 1169
## - cogerr 1 1148 1170
## - loop 1 1150 1172
## - male 1 1179 1201
##
## Step: AIC=1160
## dead ~ sah2 + male + pperf + perf + cogerr + sbp + mile + loop +
## untrt + trtdp
##
## Df Deviance AIC
## <none> 1138 1160
## - sbp 1 1140 1160
## - sah2 1 1142 1162
## - untrt 1 1143 1163
## - pperf 1 1143 1163
## - perf 1 1144 1164
## - mile 1 1147 1167
## - cogerr 1 1149 1169
## - trtdp 1 1149 1169
## - loop 1 1155 1175
## - male 1 1181 1201
##
## Call:
## glm(formula = dead ~ sah2 + male + pperf + perf + cogerr + sbp +
## mile + loop + untrt + trtdp, family = binomial(link = "logit"),
## data = sah)
##
## Deviance Residuals:
## Min 1Q Median 3Q Max
## -1.632 -0.557 -0.401 -0.281 2.559
##
## Coefficients:
## Estimate Std. Error z value Pr(>|z|)
## (Intercept) -2.831126 0.681306 -4.16 3.2e-05 ***
## sah2 0.197495 0.107006 1.85 0.06494 .
## male 1.145512 0.175180 6.54 6.2e-11 ***
## pperf -0.064669 0.028456 -2.27 0.02305 *
## perf -0.001945 0.000807 -2.41 0.01592 *
## cogerr 0.148237 0.044780 3.31 0.00093 ***
## sbp 0.005590 0.003897 1.43 0.15144
## mile -0.569343 0.192364 -2.96 0.00308 **
## loop 0.884837 0.209041 4.23 2.3e-05 ***
## untrt 0.527456 0.225157 2.34 0.01915 *
## trtdp 0.750717 0.219219 3.42 0.00062 ***
## ---
## Signif. codes: 0 '***' 0.001 '**' 0.01 '*' 0.05 '.' 0.1 ' ' 1
##
## (Dispersion parameter for binomial family taken to be 1)
##
## Null deviance: 1306.7 on 1599 degrees of freedom
## Residual deviance: 1138.2 on 1589 degrees of freedom
## AIC: 1160
##
## Number of Fisher Scoring iterations: 5
Calibration: A model is well calibrated if the observed and predicted probabilities based on the model are reasonably close.
Discrimination: A model has good discrimination if the distribution of risk scores for cases and controls separate out.
\[\hat{p}_i = \dfrac{\exp\left( \hat{\beta}_0 + \hat{\beta}_1 x_{i1} + \hat{\beta}_2 x_{i2} + \cdots + \hat{\beta}_K x_{iK} \right)}{1 + \exp\left( \hat{\beta}_0 + \hat{\beta}_1 x_{i1} + \hat{\beta}_2 x_{i2} + \cdots + \hat{\beta}_K x_{iK} \right)}\]
G
approximately equal sized groups based on the ordered \(\hat{p}\). G=10
to create deciles of increasing risk. \[ \begin{aligned} O_j &= \sum_{i\in group_j} y_i = \text{ Observed number of events in group }j\\ E_j &= \sum_{i\in group_j} \hat{p}_i = \text{ Expected number of events in group }j\\ \bar{p}_j &= \dfrac{E_j}{n_j} \;\;\;\; n_j=\text{ number of subjects in group} j=1,\ldots,G\\ \end{aligned} \]
term | estimate | p.value | conf.low | conf.high |
---|---|---|---|---|
sah2 | 1.218 | 0.065 | 0.988 | 1.504 |
male | 3.144 | 0.000 | 2.233 | 4.442 |
pperf | 0.937 | 0.023 | 0.886 | 0.991 |
perf | 0.998 | 0.016 | 0.996 | 1.000 |
cogerr | 1.160 | 0.001 | 1.062 | 1.266 |
sbp | 1.006 | 0.151 | 0.998 | 1.013 |
mile | 0.566 | 0.003 | 0.388 | 0.826 |
loop | 2.423 | 0.000 | 1.600 | 3.635 |
untrt | 1.695 | 0.019 | 1.079 | 2.612 |
trtdp | 2.119 | 0.001 | 1.367 | 3.234 |
We can test the calibration of this below:
library(ResourceSelection)
hoslem.test(sah$dead, fitted(mod.back.auto), g=10)
class(sah$dead)
table(sah$dead)
## [1] "haven_labelled"
##
## 0 1
## 1373 227
We can see that dead
is a factor without the 0 and 1 that we wanted to have for it. Basically this Stata dataset had labels and those labels are what R imported.
sah$dead <- as.numeric(sah$dead)
table(sah$dead)
##
## 0 1
## 1373 227
library(ResourceSelection)
hoslem.test(sah$dead, fitted(mod.back.auto), g=10)
##
## Hosmer and Lemeshow goodness of fit (GOF) test
##
## data: sah$dead, fitted(mod.back.auto)
## X-squared = 10, df = 8, p-value = 0.2
##
## Hosmer and Lemeshow goodness of fit (GOF) test
##
## data: sah$dead, fitted(mod.back.auto)
## X-squared = 3, df = 4, p-value = 0.5
library(ResourceSelection)
hoslem.test(sah$dead, fitted(mod.back.auto), g=6)
library(ResourceSelection)
hoslem.test(sah$dead, fitted(mod.back.auto), g=8)
##
## Hosmer and Lemeshow goodness of fit (GOF) test
##
## data: sah$dead, fitted(mod.back.auto)
## X-squared = 5, df = 6, p-value = 0.6
library(ResourceSelection)
hoslem.test(sah$dead, fitted(mod.back.auto), g=12)
##
## Hosmer and Lemeshow goodness of fit (GOF) test
##
## data: sah$dead, fitted(mod.back.auto)
## X-squared = 10, df = 10, p-value = 0.3
In each case our p-values are still showing this is a good fit.
If we consider our model from above it predicts:
Sensitivity
Specificity
library(ggplot2)
library(ROCR)
prob <- predict(mod.back.auto)
pred <- prediction(prob, sah$dead)
perf <- performance(pred, "tpr", "fpr")
# I know, the following code is bizarre. Just go with it.
auc <- performance(pred, measure = "auc")
auc <- auc@y.values[[1]]
roc.data <- data.frame(fpr=unlist(perf@x.values),
tpr=unlist(perf@y.values),
model="GLM")
ggplot(roc.data, aes(x=fpr, ymin=0, ymax=tpr)) +
geom_ribbon(alpha=0.2) + geom_abline(intercept = 0, slope = 1, colour = "gray")+
geom_line(aes(y=tpr)) +
ggtitle(paste0("ROC Curve w/ AUC=", auc))
AUC | Model Fit |
---|---|
0.5 | Random |
0.6 | Mediocre |
0.7 | Decent |
0.8 | Good |
0.9 | Excellent |