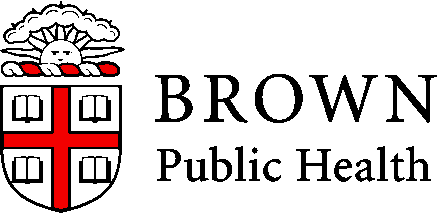
Adam J Sullivan
Assistant Professor of Biostatistics
Brown University
Name | Description |
---|---|
age | Age in years at time of Randomization |
asa | 0 - placebo, 1 - aspirin |
bmi | Body Mass Index (kg/\(m^2\)) |
hypert | 1 - Hypertensive at baseline, 0 - Not |
alcohol | 0 - less than monthly, 1 - monthly to less than daily, 2 - daily consumption |
dm | 0 = No diabetes Mellitus, 1 - diabetes Mellitus |
sbp | Systolic BP (mmHg) |
Name | Description |
---|---|
exer | 0 - No regular, 1 - Sweat at least once per week |
csmoke | 0 - Not currently, 1 - < 1 pack per day, 2 - \(\ge\) 1 pack per day |
psmoke | 0 - never smoked, 1 - former < 1 pack per day, 2 - former \(\ge\) 1 pack per day |
pkyrs | Total lifetime packs of cigarettes smoked |
crc | 0 - No colorectal Cancer, 1 - Colorectal cancer |
cayrs | Years to colorectal cancer, or death, or end of follow-up. |
library(tidyverse)
library(haven)
phscrc <- read_dta("phscrc.dta")
phscrc <- phscrc %>%
mutate(age.cat = cut(age, c(40,50,60,70, 90), right=FALSE)) %>%
mutate(alcohol.use = factor(phscrc$alcohol>0, labels=c("no", "yes")))
We then can consider the following table of information
Alcohol Users | Non-Alcohol Users | |
---|---|---|
Ages | $\dfrac{\text{Events(MI)}}{\text{Person-Years}}$ | $\dfrac{\text{Events(MI)}}{\text{Person-Years}}$ |
40-49 | $\dfrac{8}{69.723}=0.1147$ | $\dfrac{31}{208.093}=0.1490$ |
50-59 | $\dfrac{21}{172.485}=0.1217$ | $\dfrac{59}{426.540}=0.1383$ |
60-69 | $\dfrac{32}{233.063}=0.1373$ | $\dfrac{62}{410.415}=0.1511$ |
70+ | $\dfrac{20}{121.789}=0.1642$ | $\dfrac{21}{129.177}=0.1626$ |
Total | $\dfrac{81}{597.060}=0.13566$ | $\dfrac{173}{1174.225}=0.1473$ |
\[ \begin{aligned} E(Y_i) &= \mu_i = \lambda_it_i\\ \log(\mu_i) &= \log(\lambda_i) + \log(t_i)\\ &= \beta_0 + \beta_1x_{1i}+ \cdots+\beta_kx_{ki} + \log(t_i)\\ \end{aligned} \]
We can actually enter data in different ways
\[\begin{aligned} \log\left(\lambda_{x_1=0, x_2}\right) &= \beta_0 + \beta_2x_2\\ \log\left(\lambda_{x_1=1, x_2}\right) &= \beta_0 + \beta_1+ \beta_2x_2\\ \beta_1 &= \log\left(\lambda_{x_1=1, x_2}\right) - \log\left(\lambda_{x_1=0, x_2}\right)\\ &= \log\left( \dfrac{\lambda_{x_1=1, x_2}}{\lambda_{x_1=0, x_2}}\right)\\ \end{aligned}\]
\[\begin{aligned} \log\left(\lambda_{x_1, x_2}\right) &= \beta_0 + \beta_1 x_1 + \beta_2x_2\\ \log\left(\lambda_{x_1, x_2+1}\right) &= \beta_0 + \beta_1 x_1 + \beta_2(x_2+1)\\ \beta_2 &= \log\left(\lambda_{x_1, x_2+1}\right) - \log\left(\lambda_{x_1, x_2}\right)\\ &= \log\left( \dfrac{\lambda_{x_1, x_2+1}}{\lambda_{x_1, x_2}}\right)\\ \end{aligned}\]
phscrc$mean.cent.age <- phscrc$age - mean(phscrc$age, na.rm=TRUE)
fit5 <- glm(crc~alcohol.use + mean.cent.age + offset(log(cayrs)), data=phscrc, family=poisson(link='log'))
term | estimate | p.value | conf.low | conf.high |
---|---|---|---|---|
(Intercept) | 0.001 | 0.000 | 0.001 | 0.001 |
alcohol.useyes | 1.413 | 0.026 | 1.051 | 1.936 |
mean.cent.age | 1.080 | 0.000 | 1.067 | 1.093 |
\[H_0:\text{ The Model is Correctly Specified }\] \[\text{vs.}\] \[H_1:\text{ The Model is Not Correctly Specified }\]
pchisq(fit5$deviance, df=fit5$df.residual, lower.tail=FALSE)
## [1] 1
-When we deal with Poisson data we are saying that \[E(X) = Var(X)\]
We can test for this in R:
library(AER)
dispersiontest(fit5)
##
## Overdispersion test
##
## data: fit5
## z = -8, p-value = 1
## alternative hypothesis: true dispersion is greater than 1
## sample estimates:
## dispersion
## 0.984
summary(fit5)
##
## Call:
## glm(formula = crc ~ alcohol.use + mean.cent.age + offset(log(cayrs)),
## family = poisson(link = "log"), data = phscrc)
##
## Deviance Residuals:
## Min 1Q Median 3Q Max
## -0.531 -0.199 -0.148 -0.116 4.026
##
## Coefficients:
## Estimate Std. Error z value Pr(>|z|)
## (Intercept) -7.13694 0.14604 -48.87 <2e-16 ***
## alcohol.useyes 0.34583 0.15557 2.22 0.026 *
## mean.cent.age 0.07665 0.00621 12.35 <2e-16 ***
## ---
## Signif. codes: 0 '***' 0.001 '**' 0.01 '*' 0.05 '.' 0.1 ' ' 1
##
## (Dispersion parameter for poisson family taken to be 1)
##
## Null deviance: 2495.0 on 16017 degrees of freedom
## Residual deviance: 2342.6 on 16015 degrees of freedom
## (16 observations deleted due to missingness)
## AIC: 2857
##
## Number of Fisher Scoring iterations: 7
summary(fit5, dispersion=0.9841428)
##
## Call:
## glm(formula = crc ~ alcohol.use + mean.cent.age + offset(log(cayrs)),
## family = poisson(link = "log"), data = phscrc)
##
## Deviance Residuals:
## Min 1Q Median 3Q Max
## -0.531 -0.199 -0.148 -0.116 4.026
##
## Coefficients:
## Estimate Std. Error z value Pr(>|z|)
## (Intercept) -7.13694 0.14488 -49.26 <2e-16 ***
## alcohol.useyes 0.34583 0.15433 2.24 0.025 *
## mean.cent.age 0.07665 0.00616 12.45 <2e-16 ***
## ---
## Signif. codes: 0 '***' 0.001 '**' 0.01 '*' 0.05 '.' 0.1 ' ' 1
##
## (Dispersion parameter for poisson family taken to be 0.984)
##
## Null deviance: 2495.0 on 16017 degrees of freedom
## Residual deviance: 2342.6 on 16015 degrees of freedom
## (16 observations deleted due to missingness)
## AIC: 2857
##
## Number of Fisher Scoring iterations: 7