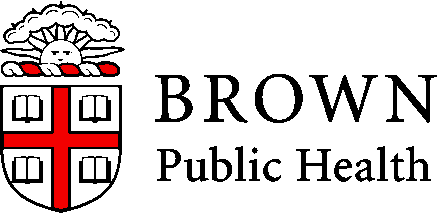
Adam J Sullivan
Assistant Professor of Biostatistics
Brown University
time to event
), where rate can depend on time in many cases. Time to relapse after end of treatment among cancer patients.
Length of stay in hospital for patients who suffered a heart attack.
The random variable of interest, \(T\) is the time to the event of interest. We then know that \(T\) is positive and by definition: \[T\ge0\]
The Survival function, \(S(t)\), is the proportion of individuals who have not experienced at event at some time \(t>0\).This is defined by: \[S(t) = \Pr(T>t)\]
###Features of \(S(t)\)
A survivor function is a sequence of probabilities up till time \(t\) \[0\le S(t)\le1, \qquad \text{ for each } t\ge0\]
At time, \(t=0\), \(S(0)=1\).
\(S(t)\) decreases as events happen over \(t\)
For large, \(t\), such as \(t=\infty\), \(S(t)\) goes to 0.
Graphical displays are a common method to display summaries survivor functions.
The Hazard function is defined as the instantaneous rate of failure at time \(t\), given that a subject as survived up until time \(t\).
It is also referred to as:
In mathematical terms:
\[\begin{aligned} h(t) &= \lim_{\Delta t\to 0}\left[ \dfrac{\Pr\left(t\le T\le t + \Delta t| T\ge t\right)}{\Delta t} \right]\\ &= \lim_{\Delta t\to 0}\left[ \dfrac{\Pr\left(t\le T\le t + \Delta t \right)}{\Delta t \Pr\left(T\ge t\right)} \right]\\ \end{aligned}\]
Note:
Definitions:
Then we have that
\[(t) = \Pr(T\le t) = 1-\Pr(T\ge t) = 1-S(t)\] \[h(t) = \dfrac{f(t)}{S(t)} = \dfrac{f(t)}{1-F(t)}\]
This means by definition:
\[\dfrac{d}{dt}S(t) = \dfrac{d}{dt}F(t) = -f(t)\]
Thus we have that
\[h(t) = \dfrac{f(t)}{S(t)} = -\dfrac{\dfrac{d}{dt}S(t))}{S(t)} = -\dfrac{d}{dt}\log\left[S(t)\right]\]
Finally
\[S(t) = \exp\left(-\int_0^t h(u)du\right) = \exp\left(-\Lambda(t)\right)\]
where \(\Lambda(t) = \int_0^th(u)du\) is the cumulative risk (hazard) function.
\[\begin{aligned} h(t)&= \lambda\\ \Lambda(t) &= \int_0^t h(u)du = \lambda t\\ S(t) &= \exp\left(-\Lambda(t)\right) = e^{-\lambda t}\\ \end{aligned}\]
Let us say the we have a constant hazard of \[h(t) = 0.01\text{ cases/person-year}\]
Then the incidence of developing disease per year:
\[ \begin{aligned} \Pr(t=10) = 0.01e^{-0.01(10)}= 0.009\text{ in year 10}\\ \Pr(t=1) = 0.01e^{-0.01(1)}= 0.010\text{ in year 1}\\ \end{aligned} \]
This means the probability of developing the disease by year 10 is \[S(t)=e^{-0.01(10)}=0.905\]
Let us say that we have a hazard rate that changes linearly with time
\[ \begin{aligned} h(t) &= 0.01*t\text{ cases/person-year}\\ h(5) &= 0.05\\ h(10)&= 0.1\\ \end{aligned} \]
Then we have the following PDF:
\[ \begin{aligned} f(t) = h(t)\exp\left(-\int_0^th(u)du\right) &= 0.01\exp\left(-\int_0^t0.01udu\right)= 0.01\exp\left(-0.005t^2\right)\\ f(5) &= 0.088\\ f(10) &= 0.06\\ \end{aligned} \]
This gives us the following survival
\[\begin{aligned} S(t) = \exp\left(-\int_0^th(u)du\right) &= \exp\left(-\int_0^t0.01udu\right)= \exp\left(-0.005t^2\right)\\ S(5) &= 0.88\\ S(10) &= 0.6\\ \end{aligned}\]
All can be used if there was no censoring.
This implies that \[\Pr(C|T=t)=\Pr(C)\]
Example when valid
Example when not valid
Many times we use nonparametric estimators - This requires that \(C\) is independent of \(T\).
We begin with an example of Remission times (time to relapse) measured in weeks for 21 leukemia patients: \[T_i = 1,1,2,2,3,4,4,5,5,8,8,8,8,11,11,12,12,15,17,22,23\]
Our goal is to estimate \(S(t)\) so we consider:
\[\hat{S}(t) = \dfrac{1}{n}\sum_{i=1}^n I(T_i\ge t)\]
For example we have that
Then since this is a binomial random variable, we can consider the variance of \(S(t)\) as \[Var\left[S(t)\right]= nS(t)\left[1-S(t)\right]\]
library(survival)
T.1 <- c(1,1,2,2,3,4,4,5,5,8,8,8,8,11,11,12,12,15,17,22,23)
event.1 <- rep(1, length(T.1))
Y <- Surv(T.1, event.1==1)
K.M <- survfit(Y~1)
summary(K.M)
## Call: survfit(formula = Y ~ 1)
##
## time n.risk n.event survival std.err lower 95% CI upper 95% CI
## 1 21 2 0.9048 0.0641 0.78754 1.000
## 2 19 2 0.8095 0.0857 0.65785 0.996
## 3 17 1 0.7619 0.0929 0.59988 0.968
## 4 16 2 0.6667 0.1029 0.49268 0.902
## 5 14 2 0.5714 0.1080 0.39455 0.828
## 8 12 4 0.3810 0.1060 0.22085 0.657
## 11 8 2 0.2857 0.0986 0.14529 0.562
## 12 6 2 0.1905 0.0857 0.07887 0.460
## 15 4 1 0.1429 0.0764 0.05011 0.407
## 17 3 1 0.0952 0.0641 0.02549 0.356
## 22 2 1 0.0476 0.0465 0.00703 0.322
## 23 1 1 0.0000 NaN NA NA
library(GGally)
plot(K.M, main="Survival Curve No Censoring", xlab="Weeks", ylab="Survival", col=c(3,4, 4))
legend(15, .9, c("Survival", "95% CI"), lty = 1:2, col=c(3,4))
We can also plot the hazard:
library(GGally)
plot(K.M, fun="cumhaz", main="Hazard Curve No Censoring", xlab="Weeks", ylab="Hazard", col=c(3,4, 4))
legend(0, 3, c("Hazard", "95% CI"), lty = 1:2, col=c(3,4))
We can also plot the hazard:
We go back to the same study as before. This was published in 1965 by Gehan. There were 21 patients in a control group and 21 patients in a drug group. They were followed to see how long in weeks before they relapsed.
We can enter these into R but this time we need to account for the censoring
T.1 <- c(1,1,2,2,3,4,4,5,5,8,8,8,8,11,11,12,12,15,17,22,23)
event.1 <- rep(1, length(T.1))
group.1 <- rep(0,length(T.1))
T.2 <- c(6,6,6,6,7,9,10,10,11,13,16,17,19,20,22,23,25,32,32,34,35)
event.2 <- c(0,1,1,1,1,0,0,1,0,1,1,0,0,0,1,1,0,0,0,0,0)
group.2 <- rep(1, length(T.2))
T.all <- c(T.1,T.2)
event.all <- c(event.1,event.2)
group.all <- c(group.1, group.2)
leuk2 <- data.frame(cbind(T.all, event.all, group.all))
The control group was easy to analyze as it had no censoring so we could calculate it by hand. However with the introduction to censoring we need a new estimator.
Let
The Kaplan-Meier estimate of survival is:
Then
\[\Pr(T_i>t_k) = \Pr(T_i>t_1|\text{at risk at }t_1) \times \cdots \times \Pr(T_i>t_k|\text{at risk at }t_k)\]
Then we have that for \(0\le t < t_1\)
\[\hat{S}(t) = 1 \]
Then for \(t_1 \le t < t_2\) we have that:
\[\begin{aligned} \hat{S}(t) &= \Pr(T>t_1)|\text{at risk at }t_1)\\ &= 1- \dfrac{d_1}{n_1}\\ &= \dfrac{n_1-d_1}{n_1}\\ \end{aligned}\]
for \(t_2 \le t < t_3\) we have that:
\[\begin{aligned} \hat{S}(t) &= \Pr(T>t_1)|\text{at risk at }t_1) \times \Pr(T>t_2)|\text{at risk at }t_2)\\ &= \hat{q}_1 \times \hat{q}_2\\ &= \left(\dfrac{n_1-d_1}{n_1}\right)\left(\dfrac{n_2-d_2}{n_2}\right)\\ &= \prod_{j=1}^2 \left(\dfrac{n_j-d_j}{n_j}\right) \end{aligned}\]
thus for any \(t_i \[\hat{S}(t) = \prod_{j=1}^i \left(\dfrac{n_j-d_j}{n_j}\right) = \prod_{j=1}^i \hat{q}_j\]
In R:
leukemia.surv <- survfit(Surv(T.all, event.all) ~ group.all , data=leuk2)
summary(leukemia.surv)
In R:
## Call: survfit(formula = Surv(T.all, event.all) ~ group.all, data = leuk2)
##
## group.all=0
## time n.risk n.event survival std.err lower 95% CI upper 95% CI
## 1 21 2 0.9048 0.0641 0.78754 1.000
## 2 19 2 0.8095 0.0857 0.65785 0.996
## 3 17 1 0.7619 0.0929 0.59988 0.968
## 4 16 2 0.6667 0.1029 0.49268 0.902
## 5 14 2 0.5714 0.1080 0.39455 0.828
## 8 12 4 0.3810 0.1060 0.22085 0.657
## 11 8 2 0.2857 0.0986 0.14529 0.562
## 12 6 2 0.1905 0.0857 0.07887 0.460
## 15 4 1 0.1429 0.0764 0.05011 0.407
## 17 3 1 0.0952 0.0641 0.02549 0.356
## 22 2 1 0.0476 0.0465 0.00703 0.322
## 23 1 1 0.0000 NaN NA NA
##
## group.all=1
## time n.risk n.event survival std.err lower 95% CI upper 95% CI
## 6 21 3 0.857 0.0764 0.720 1.000
## 7 17 1 0.807 0.0869 0.653 0.996
## 10 15 1 0.753 0.0963 0.586 0.968
## 13 12 1 0.690 0.1068 0.510 0.935
## 16 11 1 0.627 0.1141 0.439 0.896
## 22 7 1 0.538 0.1282 0.337 0.858
## 23 6 1 0.448 0.1346 0.249 0.807
suppressMessages(library(survminer))
ggsurvplot(leukemia.surv, conf.int = TRUE, risk.table = TRUE, risk.table.col="strata",
legend.labs = c("Placebo", "Treatment"),break.time.by=5)
These are based on Greenwood Formula
\[\widehat{Var}\left(\log\left[\hat{S}(t_i)\right]\right) = \sum_{j=1}^i \dfrac{d_j}{n_j(n_j-d_j)}\]
Thus a 95% CI for \(\log\left[S(t_i)\right]\) is: \[\log\left[\hat{S}(t_i)\right] \pm 1.96\sqrt{\widehat{Var}\left(\log\left[\hat{S}(t_i)\right]\right)}\] and a 95% CI for \(S(t_i)\) is
\[\hat{S}(t_i)\cdot\exp\left[\pm 1.96\sqrt{\widehat{Var}\left(\log\left[\hat{S}(t_i)\right]\right)}\right]\]
Recall
\[\Lambda(t) = \int_0^t h(u)du\] \[\text{and}\] \[S(t) = \exp\left(-\Lambda(t)\right)\]
If we consider the cumulative risk (hazard) function:
This cumulative hazard estimator is
\[\hat{\Lambda}(t) = \sum_{t_j\le t} \dfrac{d_j}{n_j}\] where \(d_j\) is the number of events observed at \(t_j\) and \(n_j\) is the number of subjects at risk at time \(t_j\)
\[\widehat{Var}\left(\hat{\Lambda}(t)\right) = \sum_{t_j\le t} \dfrac{d_j}{n^2_j}\]
leukemia.haz <- survfit(Surv(T.all, event.all) ~ group.all, type='fleming', data=leuk2)
suppressMessages(library(survminer))
ggsurvplot(leukemia.haz, conf.int = TRUE,
risk.table = TRUE, risk.table.col = "strata",
fun = "cumhaz", legend.labs = c("Placebo", "Treatment"),
break.time.by=5)
The Logrank Test is a hypothesis test for 2 or more independent samples of survival data.
The hypothesis being tested are:
\[H_o: S_1(t) = S_2(t) \text{ for all }t\] \[\text{and}\] \[H_o: S_1(t) \ne S_2(t) \text{ for some }t\]
If \(H_0\) is true then
How do we calculate this test statistic?
We have \(K\) distinct observed failure times:
\[t_1<\cdots at the \(i^{\text{th}}\) observed failure time \(t_i\):
Treatment | Died | Alive | At Risk |
---|---|---|---|
Control | \(a_i\) | \(b_i\) | \(n_{1i}\) |
Treated | \(c_i\) | \(d_i\) | \(n_{2i}\) |
total | \(m_{1i}\) | \(m_{2i}\) | \(n_i\) |
where \[\begin{aligned} n_{1i} &= \text{ numer at risk at } t_i \text{ from Control}\\ n_{2i} &= \text{ numer at risk at } t_i \text{ from Treated}\\ m_{1i} &= \text{ number of failures at } t_i\\ m_{2i} &= \text{ number surviving past } t_i\\ n_i &= \text{ total numer at risk at } t_i\\ \end{aligned}\]
This test is exactly the same as a Mantel-Haenszel test applied to \(K\) strata
\[\chi^2_{MH} = \dfrac{\left[\sum_{i=1}^K (a_i - E(a_i))\right]^2}{\sum_{i=1}^K Var(a_i)}\]
where
\[\begin{aligned} E(a_i) &= \dfrac{n_{1i}m_{1i}}{n_i}\\ Var(a_i) &= \dfrac{n_{1i}n_{2i}m_{1i}m_{2i}}{n_i^2(n_i-1)}\\ \end{aligned}\]
We compute the expectation that the null hypothesis is true and there is no difference in survival between the groups. We consider all margins fixed but \(a_i\) is random and thus we have a hypergeometric distribution.
We can run this test in R:
survdiff(Surv(T.all, event.all) ~ group.all)
## Call:
## survdiff(formula = Surv(T.all, event.all) ~ group.all)
##
## N Observed Expected (O-E)^2/E (O-E)^2/V
## group.all=0 21 21 10.7 9.77 16.8
## group.all=1 21 9 19.3 5.46 16.8
##
## Chisq= 16.8 on 1 degrees of freedom, p= 0.00004
The logrank test can also be written
\[\chi^2_{MH} = \dfrac{\sum_{i=1}^K (a_i - E(a_i))}{\sqrt{\sum_{i=1}^K Var(a_i)}} = \dfrac{D}{\sqrt{v}}\] Which is a standard normal distribution under \(H_0\).
then the stratified logrank is
\[Z_{ST} = \dfrac{\sum_{l=1}^LD_l}{\sqrt{\sum_{l=1}^LV_l}}\]
Under \(H_0\) this is also a standard normal distribution.
For this reason we use continuous distributions but ones other than normal. We will discuss a couple of these:
This is the simplest situation
We have a random variable \(T\) which is the failure time
If treatment cuts the hazard in half, the median survival time is doubled
If survival times follow an exponential distribution with the hazard \(\lambda\) then the number of events \(t\) follows a Poisson distribution with mortality rate \(\lambda\).
Thus when we use Poisson regression the estimated regression coefficients are interpreted as log-hazard ratios.
For example:
Let
Then the exponential regression model is
\[h(t|X=x) = \exp(\beta_0+\beta_1x) = \exp(\beta_0)\exp(\beta_1x)\] This means
\[\begin{aligned} \log\left[h(t|X=0)\right] &= \beta_0\\ &= \log\left( \text{ hazard among people without diabetes}\right)\\ \log\left[h(t|X=1)\right] &= \beta_0 + \beta_1\\ &= \log\left( \text{ hazard among people with diabetes}\right)\\ \beta_1 &= \log\left[h(t|X=1)\right]- \log\left[h(t|X=0)\right]\\ &= \dfrac{\log\left[h(t|X=1)\right]}{\log\left[h(t|X=0)\right]}\\ &= \log\left(\text{ hazard ratio, diabetes vs no diabetes}\right)\\ \end{aligned}\]
We typically test this assumption with a graph
\[\Lambda(t) = \int_0^th(u)du=\lambda t\] This implies that it is a straight line with slope \(\lambda\) and intercept 0.
Recall our Colorectal Cancer data and smoking:
suppressMessages(library(foreign))
phscrc <- read.dta("https://drive.google.com/uc?export=download&id=0B8CsRLdwqzbzSno1bFF4SUpVQWs")
crc.haz <- survfit(Surv(cayrs, crc) ~ csmok, data= phscrc, type='fleming')
suppressMessages(library(survminer))
ggsurvplot(crc.haz, conf.int = TRUE,
risk.table = TRUE, risk.table.col = "strata",
fun = "cumhaz", legend.labs = c("Non-smoker", "<1 pack/day", ">1 pack/day"),
break.time.by=1)
We use the Weibull many times as an alternative to the exponential
The weibull has
The model for weibull hazard is
\[h(t|X_1,\ldots,X_p ) = \lambda\gamma t ^{\gamma-1}\exp\left(\beta_0+\beta_1x_1+\cdots\beta_px_p\right)\]
Then
survreg()
Weibull is not always applicable in some settings. For example
###Why do we use parametric models?
For a time to event \(T\) and covariates \(X_1, \ldots , X_p\) the exponential regression model is:
\[h(t|X_1,\ldots, X_p) = \exp\left(\beta_0 + \beta_1x_1 + \cdots + \beta_px_p\right)\]
The baseline hazard function is defined as the hazard function when all covariates are 0
\[h(t|X_1=0,\ldots, X_p=0) = \exp\left(\beta_0 \right) = h_0(t) = h_0\]
Thus we can rewrite the model as: \[h(t|X_1,\ldots, X_p) = h_0\exp\left( \beta_1x_1 + \cdots + \beta_px_p\right)\]
This suggest that covariate effects are **multiplicative* on the constant baseline hazard, \(h_0\).
For a time to event \(T\) and covariates \(X_1, \ldots , X_p\) the Weibull regression model is:
\[h(t|X_1,\ldots, X_p) = \gamma t^{\gamma-1}\exp\left(\beta_0 + \beta_1x_1 + \cdots + \beta_px_p\right)\] with baseline hazard \[h_0(t) = \exp(\beta_0)\gamma t^{\gamma-1} = \lambda\gamma t^{\gamma-1}\] We can rewrite this as \[h(t|X_1,\ldots, X_p) = h_0(t)\exp\left(\beta_1x_1 + \cdots + \beta_px_p\right)\]
The general Proportional Hazards Model is \[h(t|X_1,\ldots, X_p) = h_0(t)\exp\left(\beta_1x_1 + \cdots + \beta_px_p\right)\]
\[\text{or}\]
\[\log\left[h(t|X_1,\ldots, X_p)\right] = \log\left[h_0(t)\right] + \beta_1x_1 + \cdots + \beta_px_p\]
where \(h_0(t)\) is the baseline hazard function and the "intercept" is \(\log\left[h_0(t)\right]\).
Let
Then
\[\begin{aligned} h(t|X=x) &= h_0(t)\exp(\beta x)\\ h(t|X=0) &= h_0(t)\\ &= \text{ baseline hazard for control group}\\ h(t|X=1) &= h_0(t)\exp(\beta)\\ &= \text{ hazard for treated group}\\ \exp(\beta) &= \dfrac{h(t|X=1)}{h(t|X=0)}\\ \end{aligned}\]
\[\begin{aligned} \log\left[h]h(t|X=0)\right] &= \log\left[h_0(t)\right]\\ \log\left[h]h(t|X=1)\right] &= \log\left[h_0(t)\right] + \beta\\ \end{aligned}\]
Recall \[S(t) = \exp\left(-\Lambda(t)\right)\] with a binary \(X\) we have that
\[\begin{aligned} \Lambda_1(t) &= \Lambda_0(t)\exp(\beta)\\ S_0(t) &= \exp(\Lambda_0(t))\\ -\log(S_0(t)) &= \Lambda_0(t)\\ \log(-\log(S_0(t))) &=\log(\Lambda_0(t))\\ \end{aligned}\]
\[\begin{aligned} S_1(t) = exp(-\Lambda_1(t)) &= \exp\left[\Lambda_0(t)\exp(\beta)\right]\\ -\log(S_1(t)) &= \Lambda_0(t)\exp(\beta)\\ \log(-\log(S_1(t))) &= \log(\Lambda_0(t)) + \beta\\ \end{aligned} \]
Thus we can see that under the assumption of proportional hazards
ggsurvplot(leukemia.surv, legend.labs = c("Placebo", "Treatment"),break.time.by=5, fun="cloglog")
cox.leukemia <- coxph(Surv(T.all, event.all) ~ group.all , data=leuk2 )
summary(cox.leukemia)
## Call:
## coxph(formula = Surv(T.all, event.all) ~ group.all, data = leuk2)
##
## n= 42, number of events= 30
##
## coef exp(coef) se(coef) z Pr(>|z|)
## group.all -1.572 0.208 0.412 -3.81 0.00014 ***
## ---
## Signif. codes: 0 '***' 0.001 '**' 0.01 '*' 0.05 '.' 0.1 ' ' 1
##
## exp(coef) exp(-coef) lower .95 upper .95
## group.all 0.208 4.82 0.0925 0.466
##
## Concordance= 0.69 (se = 0.041 )
## Rsquare= 0.322 (max possible= 0.988 )
## Likelihood ratio test= 16.4 on 1 df, p=0.00005
## Wald test = 14.5 on 1 df, p=0.0001
## Score (logrank) test = 17.2 on 1 df, p=0.00003
This would suggest that the hazard for those in a placebo group is 4.8 times that of those in the treated group.
For another example could look at Colorectal Cancer in the PHS:
crc.cox <- coxph(Surv(cayrs, crc) ~ csmok + age , data= phscrc)
summary(crc.cox)
Then we could say that for two people with the same smoking status a one year increase in age would lead to an 8.2% increase in the hazard of colorectal cancer with a 95% CI of 6.9% to 9.6%.
## Call:
## coxph(formula = Surv(cayrs, crc) ~ csmok + age, data = phscrc)
##
## n= 16018, number of events= 254
## (16 observations deleted due to missingness)
##
## coef exp(coef) se(coef) z Pr(>|z|)
## csmok 0.31715 1.37320 0.09767 3.25 0.0012 **
## age 0.07904 1.08224 0.00628 12.58 <2e-16 ***
## ---
## Signif. codes: 0 '***' 0.001 '**' 0.01 '*' 0.05 '.' 0.1 ' ' 1
##
## exp(coef) exp(-coef) lower .95 upper .95
## csmok 1.37 0.728 1.13 1.66
## age 1.08 0.924 1.07 1.10
##
## Concordance= 0.724 (se = 0.015 )
## Rsquare= 0.01 (max possible= 0.262 )
## Likelihood ratio test= 160 on 2 df, p=<2e-16
## Wald test = 163 on 2 df, p=<2e-16
## Score (logrank) test = 177 on 2 df, p=<2e-16