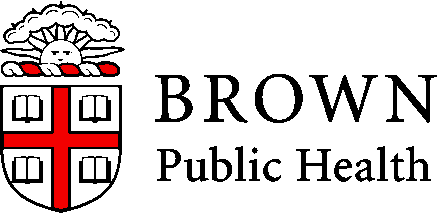
Adam J Sullivan
Assistant Professor of Biostatistics
Brown University
Many times we use nonparametric estimators - This requires that \(C\) is independent of \(T\).
We begin with an example of Remission times (time to relapse) measured in weeks for 21 leukemia patients: \[T_i = 1,1,2,2,3,4,4,5,5,8,8,8,8,11,11,12,12,15,17,22,23\]
Our goal is to estimate \(S(t)\) so we consider:
\[\hat{S}(t) = \dfrac{1}{n}\sum_{i=1}^n I(T_i\ge t)\]
For example we have that
Then since this is a binomial random variable, we can consider the variance of \(S(t)\) as \[Var\left[S(t)\right]= nS(t)\left[1-S(t)\right]\]
library(survival)
T.1 <- c(1,1,2,2,3,4,4,5,5,8,8,8,8,11,11,12,12,15,17,22,23)
event.1 <- rep(1, length(T.1))
Y <- Surv(T.1, event.1==1)
K.M <- survfit(Y~1)
summary(K.M)
## Call: survfit(formula = Y ~ 1)
##
## time n.risk n.event survival std.err lower 95% CI upper 95% CI
## 1 21 2 0.9048 0.0641 0.78754 1.000
## 2 19 2 0.8095 0.0857 0.65785 0.996
## 3 17 1 0.7619 0.0929 0.59988 0.968
## 4 16 2 0.6667 0.1029 0.49268 0.902
## 5 14 2 0.5714 0.1080 0.39455 0.828
## 8 12 4 0.3810 0.1060 0.22085 0.657
## 11 8 2 0.2857 0.0986 0.14529 0.562
## 12 6 2 0.1905 0.0857 0.07887 0.460
## 15 4 1 0.1429 0.0764 0.05011 0.407
## 17 3 1 0.0952 0.0641 0.02549 0.356
## 22 2 1 0.0476 0.0465 0.00703 0.322
## 23 1 1 0.0000 NaN NA NA
library(GGally)
plot(K.M, main="Survival Curve No Censoring", xlab="Weeks", ylab="Survival", col=c(3,4, 4))
legend(15, .9, c("Survival", "95% CI"), lty = 1:2, col=c(3,4))
We can also plot the hazard:
library(GGally)
plot(K.M, fun="cumhaz", main="Hazard Curve No Censoring", xlab="Weeks", ylab="Hazard", col=c(3,4, 4))
legend(0, 3, c("Hazard", "95% CI"), lty = 1:2, col=c(3,4))
We can also plot the hazard:
We go back to the same study as before. This was published in 1965 by Gehan. There were 21 patients in a control group and 21 patients in a drug group. They were followed to see how long in weeks before they relapsed.
We can enter these into R but this time we need to account for the censoring
T.1 <- c(1,1,2,2,3,4,4,5,5,8,8,8,8,11,11,12,12,15,17,22,23)
event.1 <- rep(1, length(T.1))
group.1 <- rep(0,length(T.1))
T.2 <- c(6,6,6,6,7,9,10,10,11,13,16,17,19,20,22,23,25,32,32,34,35)
event.2 <- c(0,1,1,1,1,0,0,1,0,1,1,0,0,0,1,1,0,0,0,0,0)
group.2 <- rep(1, length(T.2))
T.all <- c(T.1,T.2)
event.all <- c(event.1,event.2)
group.all <- c(group.1, group.2)
leuk2 <- data.frame(cbind(T.all, event.all, group.all))
The control group was easy to analyze as it had no censoring so we could calculate it by hand. However with the introduction to censoring we need a new estimator.
Let
The Kaplan-Meier estimate of survival is:
Then
\[\Pr(T_i>t_k) = \Pr(T_i>t_1|\text{at risk at }t_1) \times \cdots \times \Pr(T_i>t_k|\text{at risk at }t_k)\]
Then we have that for \(0\le t < t_1\)
\[\hat{S}(t) = 1 \]
Then for \(t_1 \le t < t_2\) we have that:
\[\begin{aligned} \hat{S}(t) &= \Pr(T>t_1)|\text{at risk at }t_1)\\ &= 1- \dfrac{d_1}{n_1}\\ &= \dfrac{n_1-d_1}{n_1}\\ \end{aligned}\]
for \(t_2 \le t < t_3\) we have that:
\[\begin{aligned} \hat{S}(t) &= \Pr(T>t_1)|\text{at risk at }t_1) \times \Pr(T>t_2)|\text{at risk at }t_2)\\ &= \hat{q}_1 \times \hat{q}_2\\ &= \left(\dfrac{n_1-d_1}{n_1}\right)\left(\dfrac{n_2-d_2}{n_2}\right)\\ &= \prod_{j=1}^2 \left(\dfrac{n_j-d_j}{n_j}\right) \end{aligned}\]
thus for any \(t_i \[\hat{S}(t) = \prod_{j=1}^i \left(\dfrac{n_j-d_j}{n_j}\right) = \prod_{j=1}^i \hat{q}_j\]
In R:
leukemia.surv <- survfit(Surv(T.all, event.all) ~ group.all , data=leuk2)
summary(leukemia.surv)
In R:
## Call: survfit(formula = Surv(T.all, event.all) ~ group.all, data = leuk2)
##
## group.all=0
## time n.risk n.event survival std.err lower 95% CI upper 95% CI
## 1 21 2 0.9048 0.0641 0.78754 1.000
## 2 19 2 0.8095 0.0857 0.65785 0.996
## 3 17 1 0.7619 0.0929 0.59988 0.968
## 4 16 2 0.6667 0.1029 0.49268 0.902
## 5 14 2 0.5714 0.1080 0.39455 0.828
## 8 12 4 0.3810 0.1060 0.22085 0.657
## 11 8 2 0.2857 0.0986 0.14529 0.562
## 12 6 2 0.1905 0.0857 0.07887 0.460
## 15 4 1 0.1429 0.0764 0.05011 0.407
## 17 3 1 0.0952 0.0641 0.02549 0.356
## 22 2 1 0.0476 0.0465 0.00703 0.322
## 23 1 1 0.0000 NaN NA NA
##
## group.all=1
## time n.risk n.event survival std.err lower 95% CI upper 95% CI
## 6 21 3 0.857 0.0764 0.720 1.000
## 7 17 1 0.807 0.0869 0.653 0.996
## 10 15 1 0.753 0.0963 0.586 0.968
## 13 12 1 0.690 0.1068 0.510 0.935
## 16 11 1 0.627 0.1141 0.439 0.896
## 22 7 1 0.538 0.1282 0.337 0.858
## 23 6 1 0.448 0.1346 0.249 0.807
suppressMessages(library(survminer))
ggsurvplot(leukemia.surv, conf.int = TRUE, risk.table = TRUE, risk.table.col="strata",
legend.labs = c("Placebo", "Treatment"),break.time.by=5)
These are based on Greenwood Formula
\[\widehat{Var}\left(\log\left[\hat{S}(t_i)\right]\right) = \sum_{j=1}^i \dfrac{d_j}{n_j(n_j-d_j)}\]
Thus a 95% CI for \(\log\left[S(t_i)\right]\) is: \[\log\left[\hat{S}(t_i)\right] \pm 1.96\sqrt{\widehat{Var}\left(\log\left[\hat{S}(t_i)\right]\right)}\] and a 95% CI for \(S(t_i)\) is
\[\hat{S}(t_i)\cdot\exp\left[\pm 1.96\sqrt{\widehat{Var}\left(\log\left[\hat{S}(t_i)\right]\right)}\right]\]
Recall
\[\Lambda(t) = \int_0^t h(u)du\] \[\text{and}\] \[S(t) = \exp\left(-\Lambda(t)\right)\]
If we consider the cumulative risk (hazard) function:
This cumulative hazard estimator is
\[\hat{\Lambda}(t) = \sum_{t_j\le t} \dfrac{d_j}{n_j}\] where \(d_j\) is the number of events observed at \(t_j\) and \(n_j\) is the number of subjects at risk at time \(t_j\)
\[\widehat{Var}\left(\hat{\Lambda}(t)\right) = \sum_{t_j\le t} \dfrac{d_j}{n^2_j}\]
leukemia.haz <- survfit(Surv(T.all, event.all) ~ group.all, type='fleming', data=leuk2)
suppressMessages(library(survminer))
ggsurvplot(leukemia.haz, conf.int = TRUE,
risk.table = TRUE, risk.table.col = "strata",
fun = "cumhaz", legend.labs = c("Placebo", "Treatment"),
break.time.by=5)
The Logrank Test is a hypothesis test for 2 or more independent samples of survival data.
The hypothesis being tested are:
\[H_o: S_1(t) = S_2(t) \text{ for all }t\] \[\text{and}\] \[H_o: S_1(t) \ne S_2(t) \text{ for some }t\]
If \(H_0\) is true then
How do we calculate this test statistic?
We have \(K\) distinct observed failure times:
\[t_1<\cdots at the \(i^{\text{th}}\) observed failure time \(t_i\):
Treatment | Died | Alive | At Risk |
---|---|---|---|
Control | \(a_i\) | \(b_i\) | \(n_{1i}\) |
Treated | \(c_i\) | \(d_i\) | \(n_{2i}\) |
total | \(m_{1i}\) | \(m_{2i}\) | \(n_i\) |
where \[\begin{aligned} n_{1i} &= \text{ numer at risk at } t_i \text{ from Control}\\ n_{2i} &= \text{ numer at risk at } t_i \text{ from Treated}\\ m_{1i} &= \text{ number of failures at } t_i\\ m_{2i} &= \text{ number surviving past } t_i\\ n_i &= \text{ total numer at risk at } t_i\\ \end{aligned}\]
This test is exactly the same as a Mantel-Haenszel test applied to \(K\) strata
\[\chi^2_{MH} = \dfrac{\left[\sum_{i=1}^K (a_i - E(a_i))\right]^2}{\sum_{i=1}^K Var(a_i)}\]
where
\[\begin{aligned} E(a_i) &= \dfrac{n_{1i}m_{1i}}{n_i}\\ Var(a_i) &= \dfrac{n_{1i}n_{2i}m_{1i}m_{2i}}{n_i^2(n_i-1)}\\ \end{aligned}\]
We compute the expectation that the null hypothesis is true and there is no difference in survival between the groups. We consider all margins fixed but \(a_i\) is random and thus we have a hypergeometric distribution.
We can run this test in R:
survdiff(Surv(T.all, event.all) ~ group.all)
## Call:
## survdiff(formula = Surv(T.all, event.all) ~ group.all)
##
## N Observed Expected (O-E)^2/E (O-E)^2/V
## group.all=0 21 21 10.7 9.77 16.8
## group.all=1 21 9 19.3 5.46 16.8
##
## Chisq= 16.8 on 1 degrees of freedom, p= 0.00004
The logrank test can also be written
\[\chi^2_{MH} = \dfrac{\sum_{i=1}^K (a_i - E(a_i))}{\sqrt{\sum_{i=1}^K Var(a_i)}} = \dfrac{D}{\sqrt{v}}\] Which is a standard normal distribution under \(H_0\).
then the stratified logrank is
\[Z_{ST} = \dfrac{\sum_{l=1}^LD_l}{\sqrt{\sum_{l=1}^LV_l}}\]
Under \(H_0\) this is also a standard normal distribution.