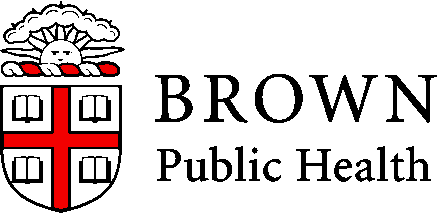
Adam J Sullivan
Assistant Professor of Biostatistics
Brown University
For this reason we use continuous distributions but ones other than normal. We will discuss a couple of these:
This is the simplest situation
We have a random variable \(T\) which is the failure time
If treatment cuts the hazard in half, the median survival time is doubled
If survival times follow an exponential distribution with the hazard \(\lambda\) then the number of events \(t\) follows a Poisson distribution with mortality rate \(\lambda\).
Thus when we use Poisson regression the estimated regression coefficients are interpreted as log-hazard ratios.
For example:
Let
Then the exponential regression model is
\[h(t|X=x) = \exp(\beta_0+\beta_1x) = \exp(\beta_0)\exp(\beta_1x)\] This means
\[\begin{aligned} \log\left[h(t|X=0)\right] &= \beta_0\\ &= \log\left( \text{ hazard among people without diabetes}\right)\\ \log\left[h(t|X=1)\right] &= \beta_0 + \beta_1\\ &= \log\left( \text{ hazard among people with diabetes}\right)\\ \beta_1 &= \log\left[h(t|X=1)\right]- \log\left[h(t|X=0)\right]\\ &= \dfrac{\log\left[h(t|X=1)\right]}{\log\left[h(t|X=0)\right]}\\ &= \log\left(\text{ hazard ratio, diabetes vs no diabetes}\right)\\ \end{aligned}\]
We typically test this assumption with a graph
\[\Lambda(t) = \int_0^th(u)du=\lambda t\] This implies that it is a straight line with slope \(\lambda\) and intercept 0.
Recall our Colorectal Cancer data and smoking:
suppressMessages(library(foreign))
phscrc <- read.dta("https://drive.google.com/uc?export=download&id=0B8CsRLdwqzbzSno1bFF4SUpVQWs")
crc.haz <- survfit(Surv(cayrs, crc) ~ csmok, data= phscrc, type='fleming')
suppressMessages(library(survminer))
ggsurvplot(crc.haz, conf.int = TRUE,
risk.table = TRUE, risk.table.col = "strata",
fun = "cumhaz", legend.labs = c("Non-smoker", "<1 pack/day", ">1 pack/day"),
break.time.by=1)
We use the Weibull many times as an alternative to the exponential
The weibull has
The model for weibull hazard is
\[h(t|X_1,\ldots,X_p ) = \lambda\gamma t ^{\gamma-1}\exp\left(\beta_0+\beta_1x_1+\cdots\beta_px_p\right)\]
Then
survreg()
Weibull is not always applicable in some settings. For example
For a time to event \(T\) and covariates \(X_1, \ldots , X_p\) the exponential regression model is:
\[h(t|X_1,\ldots, X_p) = \exp\left(\beta_0 + \beta_1x_1 + \cdots + \beta_px_p\right)\]
The baseline hazard function is defined as the hazard function when all covariates are 0
\[h(t|X_1=0,\ldots, X_p=0) = \exp\left(\beta_0 \right) = h_0(t) = h_0\]
Thus we can rewrite the model as: \[h(t|X_1,\ldots, X_p) = h_0\exp\left( \beta_1x_1 + \cdots + \beta_px_p\right)\]
This suggest that covariate effects are **multiplicative* on the constant baseline hazard, \(h_0\).
For a time to event \(T\) and covariates \(X_1, \ldots , X_p\) the Weibull regression model is:
\[h(t|X_1,\ldots, X_p) = \gamma t^{\gamma-1}\exp\left(\beta_0 + \beta_1x_1 + \cdots + \beta_px_p\right)\] with baseline hazard \[h_0(t) = \exp(\beta_0)\gamma t^{\gamma-1} = \lambda\gamma t^{\gamma-1}\] We can rewrite this as \[h(t|X_1,\ldots, X_p) = h_0(t)\exp\left(\beta_1x_1 + \cdots + \beta_px_p\right)\]
The general Proportional Hazards Model is \[h(t|X_1,\ldots, X_p) = h_0(t)\exp\left(\beta_1x_1 + \cdots + \beta_px_p\right)\]
\[\text{or}\]
\[\log\left[h(t|X_1,\ldots, X_p)\right] = \log\left[h_0(t)\right] + \beta_1x_1 + \cdots + \beta_px_p\]
where \(h_0(t)\) is the baseline hazard function and the "intercept" is \(\log\left[h_0(t)\right]\).
Let
Then
\[\begin{aligned} h(t|X=x) &= h_0(t)\exp(\beta x)\\ h(t|X=0) &= h_0(t)\\ &= \text{ baseline hazard for control group}\\ h(t|X=1) &= h_0(t)\exp(\beta)\\ &= \text{ hazard for treated group}\\ \exp(\beta) &= \dfrac{h(t|X=1)}{h(t|X=0)}\\ \end{aligned}\]
\[\begin{aligned} \log\left[h]h(t|X=0)\right] &= \log\left[h_0(t)\right]\\ \log\left[h]h(t|X=1)\right] &= \log\left[h_0(t)\right] + \beta\\ \end{aligned}\]
Recall \[S(t) = \exp\left(-\Lambda(t)\right)\] with a binary \(X\) we have that
\[\begin{aligned} \Lambda_1(t) &= \Lambda_0(t)\exp(\beta)\\ S_0(t) &= \exp(\Lambda_0(t))\\ -\log(S_0(t)) &= \Lambda_0(t)\\ \log(-\log(S_0(t))) &=\log(\Lambda_0(t))\\ \end{aligned}\]
\[ \begin{aligned} S_1(t) = exp(-\Lambda_1(t)) &= \exp\left[\Lambda_0(t)\exp(\beta)\right]\\ -\log(S_1(t)) &= \Lambda_0(t)\exp(\beta)\\ \log(-\log(S_1(t))) &= \log(\Lambda_0(t)) + \beta\\ \end{aligned} \]
Thus we can see that under the assumption of proportional hazards
ggsurvplot(leukemia.surv,
legend.labs = c("Placebo", "Treatment"),break.time.by=5,
fun="cloglog")
cox.leukemia <- coxph(Surv(T.all, event.all) ~ group.all , data=leuk2 )
summary(cox.leukemia)
## Call:
## coxph(formula = Surv(T.all, event.all) ~ group.all, data = leuk2)
##
## n= 42, number of events= 30
##
## coef exp(coef) se(coef) z Pr(>|z|)
## group.all -1.572 0.208 0.412 -3.81 0.00014 ***
## ---
## Signif. codes: 0 '***' 0.001 '**' 0.01 '*' 0.05 '.' 0.1 ' ' 1
##
## exp(coef) exp(-coef) lower .95 upper .95
## group.all 0.208 4.82 0.0925 0.466
##
## Concordance= 0.69 (se = 0.041 )
## Rsquare= 0.322 (max possible= 0.988 )
## Likelihood ratio test= 16.4 on 1 df, p=0.00005
## Wald test = 14.5 on 1 df, p=0.0001
## Score (logrank) test = 17.2 on 1 df, p=0.00003
This would suggest that the hazard for those in a placebo group is 4.8 times that of those in the treated group.
For another example could look at Colorectal Cancer in the PHS:
crc.cox <- coxph(Surv(cayrs, crc) ~ csmok + age , data= phscrc)
summary(crc.cox)
Then we could say that for two people with the same smoking status a one year increase in age would lead to an 8.2% increase in the hazard of colorectal cancer with a 95% CI of 6.9% to 9.6%.
## Call:
## coxph(formula = Surv(cayrs, crc) ~ csmok + age, data = phscrc)
##
## n= 16018, number of events= 254
## (16 observations deleted due to missingness)
##
## coef exp(coef) se(coef) z Pr(>|z|)
## csmok 0.31715 1.37320 0.09767 3.25 0.0012 **
## age 0.07904 1.08224 0.00628 12.58 <2e-16 ***
## ---
## Signif. codes: 0 '***' 0.001 '**' 0.01 '*' 0.05 '.' 0.1 ' ' 1
##
## exp(coef) exp(-coef) lower .95 upper .95
## csmok 1.37 0.728 1.13 1.66
## age 1.08 0.924 1.07 1.10
##
## Concordance= 0.724 (se = 0.015 )
## Rsquare= 0.01 (max possible= 0.262 )
## Likelihood ratio test= 160 on 2 df, p=<2e-16
## Wald test = 163 on 2 df, p=<2e-16
## Score (logrank) test = 177 on 2 df, p=<2e-16
library(SurvRegCensCov)
crc.exp <- survreg(Surv(cayrs, crc) ~ csmok + age, data= phscrc, dist='weibull')
ConvertWeibull(crc.exp)
## $vars
## Estimate SE
## lambda 0.00000664 0.00000295
## gamma 1.30451991 0.07929445
## csmok 0.31804318 0.09766224
## age 0.07916154 0.00627915
##
## $HR
## HR LB UB
## csmok 1.37 1.13 1.66
## age 1.08 1.07 1.10
##
## $ETR
## ETR LB UB
## csmok 0.784 0.675 0.910
## age 0.941 0.930 0.952