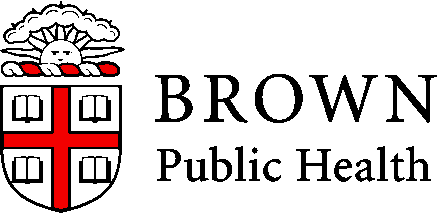
Adam J Sullivan
Assistant Professor of Biostatistics
Brown University
boot
function from the boot
package to perform the boostrappingISLR::Portfolio
data set.statistic <- function(data, index) {
x <- data$X[index]
y <- data$Y[index]
(var(y) - cov(x, y)) / (var(x) + var(y) - 2* cov(x, y))
}
portfolio <- ISLR::Portfolio
statistic(portfolio, 1:100)
## [1] 0.576
sample
to randomly select 100 observations from the range 1 to 100, with replacement. statistic(portfolio, sample(100, 100, replace = TRUE))
## [1] 0.467
boot
and supply it our original data, the function that computes the test statistic, and the number of bootstrap replicates (R
).boot()
function in Rset.seed(123)
boot(portfolio, statistic, R = 1000)
boot()
function in R##
## ORDINARY NONPARAMETRIC BOOTSTRAP
##
##
## Call:
## boot(data = portfolio, statistic = statistic, R = 1000)
##
##
## Bootstrap Statistics :
## original bias std. error
## t1* 0.576 0.0024 0.0875
boot.ci
and plot our results:set.seed(123)
result <- boot(portfolio, statistic, R = 1000)
boot.ci(result, type = "basic")
## BOOTSTRAP CONFIDENCE INTERVAL CALCULATIONS
## Based on 1000 bootstrap replicates
##
## CALL :
## boot.ci(boot.out = result, type = "basic")
##
## Intervals :
## Level Basic
## 95% ( 0.396, 0.738 )
## Calculations and Intervals on Original Scale
horsepower
to predict mpg
in our auto
data set.library(tidyverse)
auto <- as_tibble(ISLR::Auto)
statistic <- function(data, index) {
lm.fit <- lm(mpg ~ horsepower, data = data, subset = index)
coef(lm.fit)
}
statistic(auto, 1:392)
## (Intercept) horsepower
## 39.936 -0.158
boot()
set.seed(123)
boot(auto, statistic, 1000)
##
## ORDINARY NONPARAMETRIC BOOTSTRAP
##
##
## Call:
## boot(data = auto, statistic = statistic, R = 1000)
##
##
## Bootstrap Statistics :
## original bias std. error
## t1* 39.936 0.029596 0.8635
## t2* -0.158 -0.000294 0.0076
summary
function we see a difference.##
## Call:
## lm(formula = mpg ~ horsepower, data = auto)
##
## Residuals:
## Min 1Q Median 3Q Max
## -13.571 -3.259 -0.344 2.763 16.924
##
## Coefficients:
## Estimate Std. Error t value Pr(>|t|)
## (Intercept) 39.93586 0.71750 55.7 <2e-16 ***
## horsepower -0.15784 0.00645 -24.5 <2e-16 ***
## ---
## Signif. codes: 0 '***' 0.001 '**' 0.01 '*' 0.05 '.' 0.1 ' ' 1
##
## Residual standard error: 4.91 on 390 degrees of freedom
## Multiple R-squared: 0.606, Adjusted R-squared: 0.605
## F-statistic: 600 on 1 and 390 DF, p-value: <2e-16
summary
may be biased. quad.statistic <- function(data, index) {
lm.fit <- lm(mpg ~ poly(horsepower, 2), data = data, subset = index)
coef(lm.fit)
}
set.seed(1)
boot(auto, quad.statistic, 1000)
##
## ORDINARY NONPARAMETRIC BOOTSTRAP
##
##
## Call:
## boot(data = auto, statistic = quad.statistic, R = 1000)
##
##
## Bootstrap Statistics :
## original bias std. error
## t1* 23.4 0.00394 0.226
## t2* -120.1 0.11731 3.701
## t3* 44.1 0.04745 4.329
##
## Call:
## lm(formula = mpg ~ poly(horsepower, 2), data = auto)
##
## Residuals:
## Min 1Q Median 3Q Max
## -14.714 -2.594 -0.086 2.287 15.896
##
## Coefficients:
## Estimate Std. Error t value Pr(>|t|)
## (Intercept) 23.446 0.221 106.1 <2e-16 ***
## poly(horsepower, 2)1 -120.138 4.374 -27.5 <2e-16 ***
## poly(horsepower, 2)2 44.090 4.374 10.1 <2e-16 ***
## ---
## Signif. codes: 0 '***' 0.001 '**' 0.01 '*' 0.05 '.' 0.1 ' ' 1
##
## Residual standard error: 4.37 on 389 degrees of freedom
## Multiple R-squared: 0.688, Adjusted R-squared: 0.686
## F-statistic: 428 on 2 and 389 DF, p-value: <2e-16